Machine Learning E194-06
Our research aims to narrow the gap between theoretically well-understood and practically relevant machine learning.
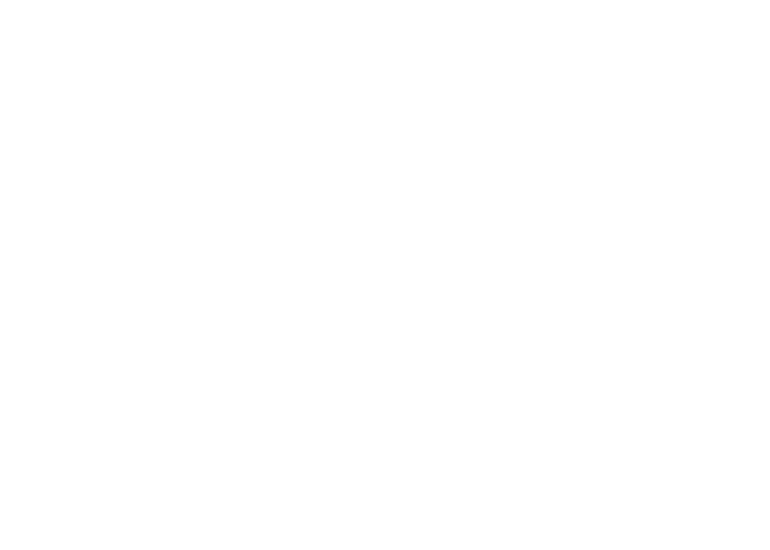
Contact
- Head: Thomas Gärtner
- Web: www.ml.tuwien.ac.at
- Phone: +43-1-58801-194602
- Location: Erzherzog-Johann-Platz 1
On This Page
About
Our research aims to narrow the gap between theoretically well-understood and practically relevant machine learning.
Research questions concern for instance:
- learning with non-conventional data, i.e., data that has no inherent representation in a table or Euclidean space
- incorporation of invariances as well as expert domain knowledge in learning algorithms
- computational, sample, query, and communication complexity of learning algorithms
- constructive machine learning scenarios such as structured output prediction
- learning with small labelled data sets and large unlabelled data sets
- adverserial learning with mistake and/or regret bounds
- parallelisation/distribution of learning algorithms
- approximation of learning algorithms
- scalability of learning algorithms
- reliability of learning algorithms
- extreme learning
- …
To demonstrate the practical effectiveness of novel learning algorithms, we apply them in Chemistry, Material Science, Electrical Engineering, Computer Games, Humanities, etc.
The research Unit Machine Learning is part of the Institute of Information Systems Engineering.
Professors
Scientific Staff
Visiting Researchers
Administrative Staff
Student Staff
External Lecturers
Courses
2024W
- Advanced Reinforcement Learning / 194.170 / VU
- Bachelor Thesis in Computer Science / 194.112 / PR
- CAIML seminar / 192.138 / SE
- Fundamentals of Digital Systems / 192.134 / VU
- Generative AI / 194.154 / VU
- Interdisciplinary Project in Data Science / 194.147 / PR
- Introduction to Machine Learning / 194.025 / VU
- Introduction to Quantum Physics: Basic Concepts and Foundations / 194.171 / VU
- Machine Learning / 184.702 / VU
- Machine Learning Algorithms and Applications / 194.101 / PR
- Modern Applications of Logic in Machine Learning / 194.173 / VU
- Privatissimum for Doctor's Thesis / 194.028 / PV
- Project in Computer Science 1 / 194.145 / PR
- Project in Computer Science 2 / 194.146 / PR
- Quantum Physics: Basic Concepts and Foundations / 194.169 / VU
- Scientific Research and Writing / 193.052 / SE
- Seminar for Master Students in Data Science / 180.772 / SE
- Seminar for PhD Students / 194.110 / SE
- Seminar in Artificial Intelligence - Theoretical Aspects of Machine Learning / 194.118 / SE
- Theoretical Foundations and Research Topics in Machine Learning / 194.100 / VU
2025S
- Algorithms and Data Structures / 186.866 / VU
- Algorithms for Data Science / 194.176 / VU
- Bachelor Thesis in Computer Science / 194.112 / PR
- CAIML seminar / 192.131 / SE
- Interdisciplinary Project in Data Science / 194.147 / PR
- Introduction to Machine Learning / 194.025 / VU
- Journal Club "Solid State Physics: Theory and Experiment" / 194.138 / SE
- Machine Learning / 184.702 / VU
- Machine Learning Algorithms and Applications / 194.101 / PR
- Modern Applications of Logic in Machine Learning / 194.173 / VU
- Orientation Bachelor with Honors of Informatics and Business Informatics / 180.767 / SE
- Project in Computer Science 1 / 194.145 / PR
- Project in Computer Science 2 / 194.146 / PR
- Reinforcement Learning / 194.163 / VU
- Scientific Research and Writing / 193.052 / SE
- Seminar for Master Students in Data Science / 180.772 / SE
- Seminar for PhD Students / 194.110 / SE
- Seminar in Artificial Intelligence - Theoretical Aspects of Machine Learning / 194.118 / SE
- Theoretical Foundations and Research Topics in Machine Learning / 194.100 / VU
Projects
-
AI and Neuroscience
2025 – 2032 / Vienna Science and Technology Fund (WWTF) -
NanoX
2024 – 2028 / Austrian Science Fund (FWF)
Publications: 208697 / 213928 -
Microscopic derivation of effective Hamiltonians
2024 – 2027 / Austrian Science Fund (FWF)
Publication: 213472 -
Doktorand/inn/enprogramm der ÖAW
2024 – 2026 / Austrian Academy of Sciences -
Modellierung und adaptive Regelung von industriellen Altholz- und Reststoffkesseln mit Neuronalen Netzen
2024 – 2025 / CONENGA Engineers GmbH -
Operational data-driven SOH estimation and prediction for maritime battery system
2024 – 2025 / AVL List GmbH -
Towards Trustworthy Recommendation Systems for Online Social Networks
2023 – 2031 / Vienna Science and Technology Fund (WWTF) -
Structured Data Learning with Generalized Similarities
2023 – 2027 / Vienna Science and Technology Fund (WWTF)
Publications: 188226 / 188931 / 188939 / 188937 / 191403 / 193749 / 193748 / 193747 / 199519 / 203813 / 203184 / 204922 / 205365 / 205196 / 208524 / 208697 / 216592 / 212560 / 213928 -
Using single atom catalysts as nanozymes in FET sensors FET
2023 – 2026 / Austrian Science Fund (FWF) -
Reliable reinforcement learning for sustainable enery systems
2023 – 2025 / Austrian Research Promotion Agency (FFG) -
Modelling Complex Structured Real Biological and Chemical Data using MachineLearning
2022 – 2023 / Austrian Exchange Service (OeAD) -
Artificial Intelligence for Advanced SAR Processing
2021 – 2023 / Austrian Research Promotion Agency (FFG)
Publication: 199834
Publications
2025
- Improving Embedded Software Development Courses: A Student Attentive Data-Driven Approach / Kissich, M., Weinbauer, K., & Baunach, M. (2025). Improving Embedded Software Development Courses: A Student Attentive Data-Driven Approach. In ECSEE ’25: Proceedings of the 6th European Conference on Software Engineering Education (pp. 171–175). https://doi.org/10.1145/3723010.3723013
- Lifted inference beyond first-order logic / Malhotra, S., Bizzaro, D., & Serafini, L. (2025). Lifted inference beyond first-order logic. Artificial Intelligence, 342, Article 104310. https://doi.org/10.1016/j.artint.2025.104310
- Disentangling real space fluctuations: The diagnostics of metal-insulator transitions beyond single-particle spectral functions / Meixner, M., Krämer, M. N., Wentzell, N., Bonetti, P. M., Andergassen, S., Toschi, A., & Schaefer, T. (2025, March 20). Disentangling real space fluctuations: The diagnostics of metal-insulator transitions beyond single-particle spectral functions [Conference Presentation]. 88. Jahrestagung der DPG und DPG-Frühjahrstagung 2025 der Sektion Kondensierte Materie, Berlin, Germany. http://hdl.handle.net/20.500.12708/213538
- Cluster extension of the DMF2RG and application to the 2d Hubbard model / Krämer, M. N., Meixner, M., Fraboulet, K., Bonetti, P. M., Vilardi, D., Wentzell, N., Schaefer, T., Toschi, A., & Andergassen, S. (2025, March 18). Cluster extension of the DMF2RG and application to the 2d Hubbard model [Conference Presentation]. 88. Jahrestagung der DPG und DPG-Frühjahrstagung 2025 der Sektion Kondensierte Materie, Berlin, Germany. http://hdl.handle.net/20.500.12708/213537
-
Interactive Knowledge-Based Kernel PCA for Solvent Selection
/
Boobier, S., Heeley, J., Gärtner, T., & Hierst, J. D. (2025). Interactive Knowledge-Based Kernel PCA for Solvent Selection. ACS Sustainable Chemistry & Engineering, 3(11), 4349–4368. https://doi.org/10.1021/acssuschemeng.4c07974
Projects: NanoX (2024–2028) / StruDL (2023–2027) -
Splitting Stump Forests: Tree Ensemble Compression for Edge Devices
/
Alkhoury, F., & Welke, P. (2025). Splitting Stump Forests: Tree Ensemble Compression for Edge Devices. In D. Pedreschi, A. Monreale, R. Guidotti, R. Pellungrini, & F. Naretto (Eds.), Discovery Science: 27th International Conference, DS 2024 Pisa, Italy, October 14–16, 2024 Proceedings, Part II (pp. 3–18). Springer Nature. https://doi.org/10.34726/8839
Download: Presentation Slides (6.95 MB)
Project: StruDL (2023–2027) - Towards Fingerprint Mosaicking Artifact Detection: A Self-Supervised Deep Learning Approach / Ruzicka, L., Alexander Spenke, Bergmann, S., Nolden, G., Kohn, B., & Heitzinger, C. (2025). Towards Fingerprint Mosaicking Artifact Detection: A Self-Supervised Deep Learning Approach. arXiv. https://doi.org/10.48550/arXiv.2501.05034
- TipSegNet: Fingertip Segmentation in Contactless Fingerprint Imaging / Ruzicka, L., Kohn, B., & Heitzinger, C. (2025). TipSegNet: Fingertip Segmentation in Contactless Fingerprint Imaging. https://doi.org/10.48550/arXiv.2501.05076
- Self-Directed Node Classification on Graphs / Sokolov, G., Thiessen, M., Akhmejanova, M., Vitale, F., & Orabona, F. (2025). Self-Directed Node Classification on Graphs. In G. Kamath & P.-L. Loh (Eds.), PMLR Proceedings of Machine Learning Research (pp. 1–31). http://hdl.handle.net/20.500.12708/213512
-
Single-boson exchange formulation of the Schwinger-Dyson equation and its application to the functional renormalization group
/
Patricolo, M., Gievers, M., Fraboulet, K., Al-Eryani, A., Heinzelmann, S., Bonetti, P., Toschi, A., Vilardi, D., & Andergassen, S. (2025). Single-boson exchange formulation of the Schwinger-Dyson equation and its application to the functional renormalization group. SciPost Physics, 18(3), Article 078. https://doi.org/10.21468/SciPostPhys.18.3.078
Project: Quantenspinsysteme P7 (2024–2027) -
SynTemp: Efficient Extraction of Graph-Based Reaction Rules from Large-Scale Reaction Databases
/
Phan, T.-L., Weinbauer, K., González Laffitte, M. E., Pan, Y., Merkle, D., Andersen, J., Fagerberg, R., Flamm, C., & Stadler, P. F. (2025). SynTemp: Efficient Extraction of Graph-Based Reaction Rules from Large-Scale Reaction Databases. Journal of Chemical Information and Modeling. https://doi.org/10.1021/acs.jcim.4c01795
Download: PDF (2.52 MB)
2024
-
Expressive Graph Representations via Homomorphisms
/
Welke, P. (2024, November 29). Expressive Graph Representations via Homomorphisms [Keynote Presentation]. LoG Paris Meetup, Paris, France. https://doi.org/10.34726/7501
Download: presentation slides (4.44 MB)
Project: StruDL (2023–2027) -
Expressive Graph Representations via Homomorphisms
/
Welke, P. (2024, November 25). Expressive Graph Representations via Homomorphisms [Presentation]. CAIML Seminar, Wien, Austria.
Project: StruDL (2023–2027) - Towards a cluster extension of DMF²RG and its application to the Hubbard model: Concepts and first results / Krämer, M. N. (2024, November 14). Towards a cluster extension of DMF2RG and its application to the Hubbard model: Concepts and first results [Presentation]. Seminar FG Theorie der stark korrelierten Quantenmaterie, Stuttgart, Germany.
- Parameter identification of shallow water waves using the generalized equal width equation and physics-informed neural networks: a conservative approximation scheme / Mohammadi, N., Abbaszadeh, M., Dehghan, M., & Heitzinger, C. (2024). Parameter identification of shallow water waves using the generalized equal width equation and physics-informed neural networks: a conservative approximation scheme. Nonlinear Dynamics. https://doi.org/10.1007/s11071-024-10497-y
- Mitigating Polarization and Disagreement Based on User Interests / Neumann, S. (2024, November 5). Mitigating Polarization and Disagreement Based on User Interests [Keynote Presentation]. MAMMOth Workshop — Part of an EU Horizon Project, Wien, Austria.
- Machine learning stochastic differential equations for the evolution of order parameters of classical many-body systems in and out of equilibrium / Carnazza, F., Carollo, F., Andergassen, S., Martius, G., Klopotek, M., & Lesanovsky, I. (2024). Machine learning stochastic differential equations for the evolution of order parameters of classical many-body systems in and out of equilibrium. MACHINE LEARNING-SCIENCE AND TECHNOLOGY, 5(4), Article 045002. https://doi.org/10.1088/2632-2153/ad7ad7
- GraphPrivatizer: Improved Structural Differential Privacy for Graph Neural Networks / Joshi, R. B., Indri, P., & Mishra, S. (2024). GraphPrivatizer: Improved Structural Differential Privacy for Graph Neural Networks. Transactions on Machine Learning Research.
-
Bandits with Abstention under Expert Advice
/
Pasteris, S., Rumi, A., Thiessen, M., Saito, S., Miyauchi, A., Vitale, F., & Herbster, M. (2024). Bandits with Abstention under Expert Advice. In NeurIPS 2024. NeurIPS 2024, Vancouver, Canada. https://doi.org/10.34726/8402
Download: Bandits with Abstention under Expert Advice (16.6 MB) - Single-boson exchange formulation of the Schwinger-Dyson equation and its application to the fRG / Patricolo, M. (2024, September 25). Single-boson exchange formulation of the Schwinger-Dyson equation and its application to the fRG [Conference Presentation]. 12th International Conference on the Exact Renormalization Group, Switzerland.
- Bosonization for fermionic fRG at weak and strong couplings / Fraboulet, K. (2024, September 25). Bosonization for fermionic fRG at weak and strong couplings [Conference Presentation]. 12th International Conference on the Exact Renormalization Group, Switzerland.
- Towards nonperturbative nonlocal correlations in the 2d Hubbard Model with the fRG / Krämer, M. N., Meixner, M., Fraboulet, K., Bonetti, P. M., Vilardi, D., Wentzell, N., Toschi, A., Schäfer, T., & Andergassen, S. (2024, September 24). Towards nonperturbative nonlocal correlations in the 2d Hubbard Model with the fRG [Poster Presentation]. 12th International Conference on the Exact Renormalization Group, Switzerland. http://hdl.handle.net/20.500.12708/202011
- Sublinear-Time Opinion Estimation / Neumann, S. (2024, September 24). Sublinear-Time Opinion Estimation [Conference Presentation]. Dagstuhl Seminar 24391: Statistical and Probabilistic Methods in Algorithmic Data Analysis, Germany.
- fRG Analysis and Fluctuation Diagnostics of the Hubbard-Holstein Model / Al-Eryani, A., Andergassen, S., & Scherer, M. (2024, September 24). fRG Analysis and Fluctuation Diagnostics of the Hubbard-Holstein Model [Poster Presentation]. 12th International Conference on the Exact Renormalization Group, Switzerland.
- Fluctuation Diagnostics of the influence of Einstein Phonons on Electronic Orders in the Normal State / Al-Eryani, A., Andergassen, S., & Scherer, M. (2024, September 18). Fluctuation Diagnostics of the influence of Einstein Phonons on Electronic Orders in the Normal State [Poster Presentation]. Autumn School on Correlated Electrons: Correlations and Phase Transitions, Germany. http://hdl.handle.net/20.500.12708/203072
- Development and external validation of temporal fusion transformer models for continuous intraoperative blood pressure forecasting / Kapral, L., Dibiasi, C., Jeremic, N., Bartos, S., Behrens, S., Bilir, A., Heitzinger, C., & Kimberger, O. (2024). Development and external validation of temporal fusion transformer models for continuous intraoperative blood pressure forecasting. EClinicalMedicine, 75, Article 102797. https://doi.org/10.1016/j.eclinm.2024.102797
- Understanding Domain-Size Generalization in Markov Logic Networks / Chen, F., Weitkämper, F., & Malhotra, S. (2024). Understanding Domain-Size Generalization in Markov Logic Networks. In Machine Learning and Knowledge Discovery in Databases. Research Track: European Conference, ECML PKDD 2024, Vilnius, Lithuania, September 9–13, 2024, Proceedings, Part VII (pp. 297–314). https://doi.org/10.1007/978-3-031-70368-3_18
-
Scalable Interactive Data Visualization
/
Chen, F., & Gärtner, T. (2024). Scalable Interactive Data Visualization. In A. Bifet, P. Daniusis, & J. Davis (Eds.), Machine Learning and Knowledge Discovery in Databases. Research Track and Demo Track : European Conference, ECML PKDD 2024, Vilnius, Lithuania, September 9–13, 2024, Proceedings, Part VIII (pp. 429–433). Springer. https://doi.org/10.1007/978-3-031-70371-3_34
Project: StruDL (2023–2027) - Adapting to the "Open World": The Utility of Hybrid Hierarchical Reinforcement Learning and Symbolic Planning / Lorang, P., Horvath, H., Kietreiber, T., Zips, P., Heitzinger, C., & Scheutz, M. (2024). Adapting to the “Open World”: The Utility of Hybrid Hierarchical Reinforcement Learning and Symbolic Planning. In 2024 IEEE International Conference on Robotics and Automation (ICRA) (pp. 508–514). https://doi.org/10.1109/ICRA57147.2024.10611594
- Compressing the two-particle Green’s function using wavelets: Theory and application to the Hubbard atom / Moghadas, E., Dräger, N., Toschi, A., Zang, J., Medvidović, M., Kiese, D., Millis, A. J., Sengupta, A., Andergassen, S., & Di Sante, D. (2024). Compressing the two-particle Green’s function using wavelets: Theory and application to the Hubbard atom. European Physical Journal Plus, 139, Article 700. https://doi.org/10.1140/epjp/s13360-024-05403-9
- Quantitative fRG and bosonization for the description of many-electron systems at weak and strong couplings / Fraboulet, K. (2024, July 25). Quantitative fRG and bosonization for the description of many-electron systems at weak and strong couplings [Presentation]. Seminar at the MPI-FKF, Germany.
- Non-local interactions from weak to strong coupling: a functional RG description / Andergassen, S. (2024, July 23). Non-local interactions from weak to strong coupling: a functional RG description [Keynote Presentation]. QUAST Colloquium, Austria.
- Machine learning dynamics of order parameters / Carnazza, F., Carollo, F., Klopotek, M., Martius, G., Andergassen, S., & Lesanovsky, I. (2024, July 9). Machine learning dynamics of order parameters [Poster Presentation]. 6th Cluster Conference “Machine Learning in Science” 2024, Germany. http://hdl.handle.net/20.500.12708/199767
- Distillation based Robustness Verification with PAC Guarantees / Indri, P., Blohm, P., Athavale, A., Bartocci, E., Weissenbacher, G., Maffei, M., Nickovic, D., Gärtner, T., & Malhotra, S. (2024). Distillation based Robustness Verification with PAC Guarantees. In International Conference on Machine Learning 2024 - Next Generation of AI Safety Workshop. International Conference on Machine Learning 2024 - Next Generation of AI Safety Workshop, Vienna, Austria. http://hdl.handle.net/20.500.12708/200890
-
Logical Distillation of Graph Neural Networks
/
Pluska, A., Welke, P., Gärtner, T., & Malhotra, S. (2024). Logical Distillation of Graph Neural Networks. In ICML 2024 Workshop on Mechanistic Interpretability. ICML 2024 Workshop on Mechanistic Interpretability, Vienna, Austria. https://doi.org/10.34726/7099
Download: PDF (309 KB)
Project: StruDL (2023–2027) - Bandits with Abstention under Expert Advice / Pasteris, S., Rumi, A., Thiessen, M., Saito, S., Miyauchi, A., Vitale, F., & Herbster, M. (2024, June 17). Bandits with Abstention under Expert Advice [Poster Presentation]. ICML 2024 Workshop: Foundations of Reinforcement Learning and Control -- Connections and Perspectivesing (ICML 2024), Vienna, Austria. http://hdl.handle.net/20.500.12708/199835
- Sublinear-Time Clustering Oracle for Signed Graphs / Neumann, S. (2024, May 16). Sublinear-Time Clustering Oracle for Signed Graphs [Conference Presentation]. SIAM Linear Algebra 2024, Paris, France.
- Sublinear-Time Opinion Estimation in the Friedkin--Johnsen Model / Neumann, S., Dong, Y., & Peng, P. (2024). Sublinear-Time Opinion Estimation in the Friedkin--Johnsen Model. In Proceedings of the ACM on Computer Graphics and Interactive Techniques (pp. 2563–2571). Association for Computing Machinery (ACM). https://doi.org/10.1145/3589334.3645572
-
Weisfeiler and Leman go Loopy: A New Hierarchy for Graph Representational Learning
/
Paolino, R., Maskey, S., Welke, P., & Kutyniok, G. (2024, May 11). Weisfeiler and Leman go Loopy: A New Hierarchy for Graph Representational Learning [Poster Presentation]. ICLR 2024 Workshop Bridging the Gap Between Practice and Theory in Deep Learning, Austria. https://doi.org/10.34726/6959
Download: poster (680 KB)
Project: StruDL (2023–2027) -
The Expressive Power of Path-Based Graph Neural Networks
/
Graziani, C., Drucks, T., Jogl, F., Bianchini, M., Scarselli, F., & Gärtner, T. (2024). The Expressive Power of Path-Based Graph Neural Networks. In Proceedings of the 41st International Conference on Machine Learning. International Conference on Machine Learning (2024), Vienna, Austria. PMLR. http://hdl.handle.net/20.500.12708/199519
Download: PDF (1.68 MB)
Project: StruDL (2023–2027) - Toward Synthetic Physical Fingerprint Targets / Ruzicka, L., Strobl, B., Bergmann, S., Nolden, G., Michalsky, T., Domscheit, C., Priesnitz, J., Blümel, F., Kohn, B., & Heitzinger, C. (2024). Toward Synthetic Physical Fingerprint Targets. Sensors, 24(9), Article 2847. https://doi.org/10.3390/s24092847
- Inferring interpretable dynamical generators of local quantum observables from projective measurements through machine learning / Cemin, G., Carnazza, F., Andergassen, S., Martius, G., Carollo, F., & Lesanovsky, I. (2024). Inferring interpretable dynamical generators of local quantum observables from projective measurements through machine learning. Physical Review Applied, 21(4), Article L041001. https://doi.org/10.1103/PhysRevApplied.21.L041001
- Towards nonperturbative nonlocal correlations in the 2d Hubbard Model with the fRG / Krämer, M., Meixner, M., Fraboulet, K., Bonetti, P. M., Vilardi, D., Wentzell, N., Schäfer, T., Toschi, A., & Andergassen, S. (2024, March 22). Towards nonperturbative nonlocal correlations in the 2d Hubbard Model with the fRG [Conference Presentation]. DPG Verhandlungen der Deutschen Physikalischen Gesellschaft 2024, Freiburg, Germany. http://hdl.handle.net/20.500.12708/196105
- Engineering the speedup of quantum tunneling in Josephson systems via dissipation / Hauff, J., Ankerhold, J., Rastelli, G., Belzig, W., Andergassen, S., & Maile, D. (2024, March 20). Engineering the speedup of quantum tunneling in Josephson systems via dissipation [Conference Presentation]. DPG Verhandlungen der Deutschen Physikalischen Gesellschaft 2024, Freiburg, Germany. http://hdl.handle.net/20.500.12708/196025
- Improving Control of Energy Systems With Reinforcement Learning: Application to a Reversible Pump Turbine / Freiin von Tubeuf, C. S., aus der Schmitten, J., Hofmann, R., Heitzinger, C., & Birkelbach, F. (2024). Improving Control of Energy Systems With Reinforcement Learning: Application to a Reversible Pump Turbine. In Proceedings of the ASME 2024 18th International Conference on Energy Sustainability ES2024. 18th International Conference on Energy Sustainability (ASME ES 2024), Anaheim, United States of America (the). https://doi.org/10.1115/ES2024-122475
- A Short Introduction to Artificial Intelligence: Methods, Success Stories, and Current Limitations / Clemens Heitzinger, & Stefan Woltran. (2024). A Short Introduction to Artificial Intelligence: Methods, Success Stories, and Current Limitations. In H. Werthner, C. Ghezzi, & J. Kramer (Eds.), Introduction to Digital Humanism : A Textbook (pp. 135–149). Springer. https://doi.org/10.1007/978-3-031-45304-5_9
- Weisfeiler and Leman Go Loopy: A New Hierarchy for Graph Representational Learning / Paolino, R., Maskey, S., Welke, P., & Kutyniok, G. (2024). Weisfeiler and Leman Go Loopy: A New Hierarchy for Graph Representational Learning. In 38th Conference on Neural Information Processing Systems (NeurIPS 2024). NeurIPS 2024, Vancouver, Canada. http://hdl.handle.net/20.500.12708/210873
- Weisfeiler and Leman Go Loopy: A New Hierarchy for Graph Representational Learning / Paolino, R., Maskey, S., Welke, P., & Kutyniok, G. (2024). Weisfeiler and Leman Go Loopy: A New Hierarchy for Graph Representational Learning. In Advances in Neural Information Processing Systems 37 (NeurIPS 2024) (pp. 120780–120831). Curran Associates, Inc. http://hdl.handle.net/20.500.12708/211125
-
The Impact of External Sources on the Friedkin–Johnsen Model
/
Out, C., Tu, S., Neumann, S., & Zehmakan, A. N. (2024). The Impact of External Sources on the Friedkin–Johnsen Model. In CIKM ’24: Proceedings of the 33rd ACM International Conference on Information and Knowledge Management (pp. 1815–1824). ACM. https://doi.org/10.1145/3627673.3679780
Download: PDF (2.69 MB) -
Is Expressivity Essential for the Predictive Performance of Graph Neural Networks?
/
Jogl, F., Welke, P., & Gärtner, T. (2024). Is Expressivity Essential for the Predictive Performance of Graph Neural Networks? In NeurIPS 2024 Workshop on Scientific Methods for Understanding Deep Learning. NeurIPS 2024 The Thirty-Eighth Annual Conference on Neural Information Processing Systems, Vancouver, Canada.
Project: StruDL (2023–2027) -
A Theory of Interpretable Approximations
/
Bressan, M., Cesa-Bianchi, N., Esposito, E., Mansour, Y., Moran, S., & Thiessen, M. (2024). A Theory of Interpretable Approximations. In S. Agrawal & A. Roth (Eds.), Proceedings of Thirty Seventh Conference on Learning Theory. http://hdl.handle.net/20.500.12708/199886
Download: PDF (344 KB) -
Partial Imaginary Transition State (ITS) Graphs: A Formal Framework for Research and Analysis of Atom-to-Atom Maps of Unbalanced Chemical Reactions and Their Completions
/
González Laffitte, M. E., Weinbauer, K., Phan, T.-L., Beier, N., Domschke, N., Flamm, C., Gatter, T., Merkle, D., & Stadler, P. F. (2024). Partial Imaginary Transition State (ITS) Graphs: A Formal Framework for Research and Analysis of Atom-to-Atom Maps of Unbalanced Chemical Reactions and Their Completions. SYMMETRY-BASEL, 16(9), Article 1217. https://doi.org/10.3390/sym16091217
Download: PDF (1.42 MB) - Mott transition and pseudogap of the square-lattice Hubbard model: Results from center-focused cellular dynamical mean-field theory / Meixner, M., Menke, H., Klett, M., Heinzelmann, S., Andergassen, S., Hansmann, P., & Schäfer, T. (2024). Mott transition and pseudogap of the square-lattice Hubbard model: Results from center-focused cellular dynamical mean-field theory. SciPost Physics, 16(2), Article 059. https://doi.org/10.21468/SciPostPhys.16.2.059
- Simple and Effective Transfer Learning for Neuro-Symbolic Integration / Daniele, A., Campari, T., Malhotra, S., & Serafini, L. (2024). Simple and Effective Transfer Learning for Neuro-Symbolic Integration. In T. R. Besold, A. S. d’Avila Garcez, E. Jimenez-Ruiz, R. Confalonieri, P. Madhyastha, & B. Wagner (Eds.), Neural-Symbolic Learning and Reasoning (pp. 166–179). https://doi.org/10.34726/7321
-
Modeling the Impact of Timeline Algorithms on Opinion Dynamics Using Low-rank Updates
/
Zhou, T., Neumann, S., Garimella, K., & Gionis, A. (2024). Modeling the Impact of Timeline Algorithms on Opinion Dynamics Using Low-rank Updates. In Proceedings of the ACM Web Conference 2024 (pp. 2694–2702). ACM. https://doi.org/10.1145/3589334.3645714
Download: PDF (1.4 MB) -
Efficient Algorithms for Learning Monophonic Halfspaces in Graphs
/
Bressan, M., Esposito, E., & Thiessen, M. (2024). Efficient Algorithms for Learning Monophonic Halfspaces in Graphs. In Proceedings of Thirty Seventh Conference on Learning Theory. 37th Annual Conference on Learning Theory, Edmonton, Canada. http://hdl.handle.net/20.500.12708/199834
Download: PDF (383 KB)
Project: AI4SAR (2021–2023) -
Reaction rebalancing: a novel approach to curating reaction databases
/
Phan, T.-L., Klaus Weinbauer, Thomas Gärtner, Merkle, D., Andersen, J., Fagerberg, R., & Stadler, P. F. (2024). Reaction rebalancing: a novel approach to curating reaction databases. Journal of Cheminformatics, 16(1), Article 82. https://doi.org/10.1186/s13321-024-00875-4
Project: StruDL (2023–2027) -
Logical Distillation of Graph Neural Networks
/
Pluska, A., Welke, P., Gärtner, T., & Malhotra, S. (2024). Logical Distillation of Graph Neural Networks. In P. Marquis, M. Ortiz, & M. Pagnucco (Eds.), Proceedings of the 21st International Conference on Principles of Knowledge Representation and Reasoning (pp. 920–930). IJCAI Organization. https://doi.org/10.24963/kr.2024/86
Download: PDF (213 KB)
Projects: NanoX (2024–2028) / StruDL (2023–2027)
2023
- Improving sensor interoperability between contactless and contact-based fingerprints using pose correction and unwarping / Ruzicka, L., Dominik Söllinger, Kohn, B., Heitzinger, C., Uhl, A., & Strobl, B. (2023). Improving sensor interoperability between contactless and contact-based fingerprints using pose correction and unwarping. IET Biometrics, 2023, Article 7519499. https://doi.org/10.1049/2023/7519499
-
Maximally Expressive GNNs for Outerplanar Graphs
/
Bause, F., Jogl, F., Indri, P., Drucks, T., Penz, D., Kriege, N., Gärtner, T., Welke, P., & Thiessen, M. (2023). Maximally Expressive GNNs for Outerplanar Graphs. In NeurIPS 2023 Workshop: New Frontiers in Graph Learning. NeurIPS 2023 Workshop: New Frontiers in Graph Learning, New Orleans, LA, United States of America (the). OpenReview.net. https://doi.org/10.34726/5433
Download: PDF (880 KB)
Project: StruDL (2023–2027) -
Extending Graph Neural Networks with Global Features
/
Brasoveanu, A. D., Jogl, F., Welke, P., & Thiessen, M. (2023, December 1). Extending Graph Neural Networks with Global Features [Poster Presentation]. Learning-on-Graphs Conference 2023: Local Meetup, München, Germany. https://doi.org/10.34726/5343
Downloads: Paper (365 KB) / Poster (289 KB) -
Maximally Expressive GNNs for Outerplanar Graphs
/
Bause, F., Jogl, F., Indri, P., Drucks, T., Penz, D., Kriege, N., Gärtner, T., Welke, P., & Thiessen, M. (2023, December 1). Maximally Expressive GNNs for Outerplanar Graphs [Poster Presentation]. Learning-on-Graphs Conference 2023: Local Meetup, München, Germany. https://doi.org/10.34726/5344
Downloads: Paper (880 KB) / Poster (422 KB)
Project: StruDL (2023–2027) -
Extending Graph Neural Networks with Global Features
/
Brasoveanu, A. D., Jogl, F., Welke, P., & Thiessen, M. (2023, November 27). Extending Graph Neural Networks with Global Features [Poster Presentation]. Learning on Graphs Conference 2023, Austria. https://doi.org/10.34726/5281
Download: Camera-ready full paper (365 KB) -
Maximally Expressive GNNs for Outerplanar Graphs
/
Bause, F., Jogl, F., Welke, P., & Thiessen, M. (2023). Maximally Expressive GNNs for Outerplanar Graphs. In The Second Learning on Graphs Conference (LoG 2023). Second Learning on Graphs Conference (LoG 2023), Austria. OpenReview.net. https://doi.org/10.34726/5434
Download: PDF (541 KB)
Project: StruDL (2023–2027) -
ModelRevelator: Fast phylogenetic model estimation via deep learning
/
Burgstaller-Muehlbacher, S., Crotty, S., Schmidt, H., Reden, F., Drucks, T., & von Haeseler, A. (2023). ModelRevelator: Fast phylogenetic model estimation via deep learning. Molecular Phylogenetics and Evolution, 188, Article 107905. https://doi.org/10.1016/j.ympev.2023.107905
Download: PDF (5.18 MB) -
No PAIN no Gain: More Expressive GNNs with Paths
/
Graziani, C., Drucks, T., Bianchini, M., Scarselli, F., & Gärtner, T. (2023). No PAIN no Gain: More Expressive GNNs with Paths. In NeurIPS 2023 Workshop: New Frontiers in Graph Learning. NeurIPS 2023 Workshop: New Frontiers in Graph Learning, New Orleans, LA, United States of America (the). OpenReview.net. https://doi.org/10.34726/5429
Download: PDF (1.01 MB) - Expressivity-Preserving GNN Simulation / Jogl, F., Thiessen, M., & Gärtner, T. (2023). Expressivity-Preserving GNN Simulation. In Advances in Neural Information Processing Systems. 37th Annual Conference on Neural Information Processing Systems (NeurIPS 2023), New Orleans, United States of America (the).
- Correlations on all length scales in the Hubbard model and beyond / Andergassen, S. (2023, September 21). Correlations on all length scales in the Hubbard model and beyond [Conference Presentation]. PHD-WORKSHOP FOR 5413, Germany.
- fRG analysis of the extended Hubbard model - SBE fluctuation diagnostics of screening / Al-Eryani, A., Heinzelmann, S., & Andergassen, S. (2023, September 18). fRG analysis of the extended Hubbard model - SBE fluctuation diagnostics of screening [Poster Presentation]. Autumn School on Correlated Electrons: Orbital Physics in Correlated Matter, Germany. http://hdl.handle.net/20.500.12708/188844
- Single-boson-exchange functional renormalization group and its application to the Hubbard model / Fraboulet, K., & Andergassen, S. (2023, September 6). Single-boson-exchange functional renormalization group and its application to the Hubbard model [Conference Presentation]. numErical MEthods for fRG in condENsed maTter, Germany. http://hdl.handle.net/20.500.12708/188320
- Modular fRG Code for Lattice Fermion Systems: Challenges, Strategies and Ideas / Al-Eryani, A., & Andergassen, S. (2023, September 6). Modular fRG Code for Lattice Fermion Systems: Challenges, Strategies and Ideas [Conference Presentation]. numErical MEthods for fRG in condENsed maTter, Germany. http://hdl.handle.net/20.500.12708/188319
- Cluster Extension of DMF²RG / Krämer, M., & Andergassen, S. (2023, September 5). Cluster Extension of DMF2RG [Poster Presentation]. numErical MEthods for fRG in condENsed maTter, Germany. http://hdl.handle.net/20.500.12708/188317
- Single Boson Exchange fRG for Extended Hubbard Interactions / Al-Eryani, A., Scherer, M., & Andergassen, S. (2023, September 5). Single Boson Exchange fRG for Extended Hubbard Interactions [Poster Presentation]. numErical MEthods for fRG in condENsed maTter, Germany. http://hdl.handle.net/20.500.12708/188318
- Functional Renormalization Group Analysis of the Pseudogap Opening in the Hubbard Model / Patricolo, M., & Andergassen, S. (2023, September 5). Functional Renormalization Group Analysis of the Pseudogap Opening in the Hubbard Model [Poster Presentation]. numErical MEthods for fRG in condENsed maTter, Germany.
- Cluster extension of DMF2RG / Krämer, M., Fraboulet, K., Vilardi, D., Bonetti, P. M., Meixner, M., Schäfer, T., & Andergassen, S. (2023, July 24). Cluster extension of DMF2RG [Poster Presentation]. Workshop “Correlations in Novel Quantum Materials” 2023, Stuttgart, Germany. http://hdl.handle.net/20.500.12708/187904
- Single-boson-exchange functional renormalization group and its application to the Hubbard model / Fraboulet, K., Al-Eryani, A., Andergassen, S., & Heinzelmann, S. (2023, July 24). Single-boson-exchange functional renormalization group and its application to the Hubbard model [Poster Presentation]. Workshop “Correlations in Novel Quantum Materials” 2023, Germany. http://hdl.handle.net/20.500.12708/187907
- The Mott metal-insulator transition in the two-dimensional Hubbard model - a cellular dynamical mean-field study on large clusters / Meixner, M., Klett, M., Heinzelmann, S., Wentzell, N., Hansmann, P., Andergassen, S., & Schäfer, T. (2023, July 24). The Mott metal-insulator transition in the two-dimensional Hubbard model - a cellular dynamical mean-field study on large clusters [Poster Presentation]. Workshop “Correlations in Novel Quantum Materials” 2023, Germany. http://hdl.handle.net/20.500.12708/187908
- Efficient fRG flow equations for extended interactions and an application to the square and triangular lattices / Al-Eryani, A., Heinzelmann, S., Fraboulet, K., Scherer, M., & Andergassen, S. (2023, July 24). Efficient fRG flow equations for extended interactions and an application to the square and triangular lattices [Poster Presentation]. Workshop “Correlations in Novel Quantum Materials” 2023, Germany. http://hdl.handle.net/20.500.12708/188221
- Pseudogap opening in the Hubbard model at strong coupling / Patricolo, M., Vilardi, D., Heinzelmann, S., Andergassen, S., & Bonetti, V. (2023, July 24). Pseudogap opening in the Hubbard model at strong coupling [Poster Presentation]. Workshop “Correlations in Novel Quantum Materials” 2023, Germany. http://hdl.handle.net/20.500.12708/187905
-
Hidden Schema Networks
/
Sanchez, R., Conrads, L., Welke, P., Cvejoski, K., & Ojeda, C. (2023). Hidden Schema Networks. In Proceedings of the 61st Annual Meeting of the Association for Computational Linguistics (Volume 1: Long Papers) (pp. 4764–4798). Association for Computational Linguistics. https://doi.org/10.18653/v1/2023.acl-long.263
Project: StruDL (2023–2027) -
A New Aligned Simple German Corpus
/
Toborek, V., Busch, M., Boßert, M., Bauckhage, C., & Welke, P. (2023). A New Aligned Simple German Corpus. In Proceedings of the 61st Annual Meeting of the Association for Computational Linguistics (pp. 11393–11412). Association for Computational Linguistics. https://doi.org/10.18653/v1/2023.acl-long.638
Project: StruDL (2023–2027) - AFEM for the fractional Laplacian / Melenk, J. M., Bahr, B., Faustmann, M., Parvizi, M., & Praetorius, D. (2023, June 12). AFEM for the fractional Laplacian [Conference Presentation]. Foundations of Computational Mathematics (FoCM 2023), Paris, France.
- Machine learning dynamics of quantum systems / Andergassen, S. (2023, May 8). Machine learning dynamics of quantum systems [Presentation]. TACO Colloquium, Austria.
- Pseudogap opening in the Hubbard model at strong coupling / Patricolo, M., Heinzelmann, S., Bonetti, P. M., Vilardi, D., & Andergassen, S. (2023, May 4). Pseudogap opening in the Hubbard model at strong coupling [Poster Presentation]. Exploring New Topics with Functional Renormalisation, Germany. http://hdl.handle.net/20.500.12708/187802
-
Can stochastic weight averaging improve generalization in private learning?
/
Patrick Indri, Tamara Drucks, & Gärtner, T. (2023). Can stochastic weight averaging improve generalization in private learning? In ICLR 2023 Workshop on Trustworthy and Reliable Large-Scale Machine Learning Models. ICLR 2023 Workshop on Trustworthy and Reliable Large-Scale Machine Learning Models, Kigali, Rwanda. https://doi.org/10.34726/5349
Download: Main paper (366 KB) - Machine learning dynamics of quantum systems / Andergassen, S. (2023, April 13). Machine learning dynamics of quantum systems [Conference Presentation]. Machine Learning and (Quantum) Physics Workshop 2023, Obergurgl, Austria.
- Krein support vector machine classification of antimicrobial peptides / Redshaw, J., Ting, D. S. J., Brown, A., Hirst, J. D., & Gärtner, T. (2023). Krein support vector machine classification of antimicrobial peptides. Digital Discovery. https://doi.org/10.1039/D3DD00004D
- Development of a reinforcement learning algorithm to optimize corticosteroid therapy in critically ill patients with sepsis / Bologheanu, R., Kapral, L., Laxar, D., Maleczek, M., Dibiasi, C., Zeiner, S., Agibetov, A., Ercole, A., Thoral, P., Elbers, P., Clemens Heitzinger, & Kimberger, O. (2023). Development of a reinforcement learning algorithm to optimize corticosteroid therapy in critically ill patients with sepsis. Journal of Clinical Medicine, 12(4), Article 1513. https://doi.org/10.3390/jcm12041513
-
An Empirical Evaluation of the Rashomon Effect in Explainable Machine Learning
/
Müller, S., Toborek, V., Beckh, K., Jakobs, M., Bauckhage, C., & Welke, P. (2023). An Empirical Evaluation of the Rashomon Effect in Explainable Machine Learning. In D. Koutra, C. Plant, M. Gomez Rodriguez, E. Baralis, & F. Bonchi (Eds.), Machine Learning and Knowledge Discovery in Databases: Research Track : European Conference, ECML PKDD 2023, Turin, Italy, September 18–22, 2023, Proceedings, Part III (pp. 462–478). Springer. https://doi.org/10.1007/978-3-031-43418-1_28
Project: StruDL (2023–2027) -
Graph Pooling Provably Improves Expressivity
/
Lachi, V., Moallemy-Oureh, A., Roth, A., & Welke, P. (2023). Graph Pooling Provably Improves Expressivity. In NeurIPS 2023 Workshop: New Frontiers in Graph Learning. NeurIPS 2023 Workshop: New Frontiers in Graph Learning, New Orleans, LA, United States of America (the). OpenReview.net. https://doi.org/10.34726/5432
Downloads: PDF (245 KB) / Poster (210 KB)
Project: StruDL (2023–2027) - Deep Symbolic Learning: Discovering Symbols and Rules from Perceptions / Daniele, A., Campari, T., Malhotra, S., & Serafini, L. (2023). Deep Symbolic Learning: Discovering Symbols and Rules from Perceptions. In Proceedings of the Thirty-Second International Joint Conference on Artificial Intelligence (IJCAI-23) (pp. 3597–3605). International Joint Conferences on Artificial Intelligence. https://doi.org/10.24963/ijcai.2023/400
- Retention is All You Need / Mohiuddin, K., Alam, M. A., Alam, M. M., Welke, P., Martin, M., Lehmann, J., & Vahdati, S. (2023). Retention is All You Need. In Proceedings of the 32nd ACM International Conference on Information and Knowledge Management (pp. 4752–4758). https://doi.org/10.1145/3583780.3615497
-
Extending Graph Neural Networks with Global Features
/
Brasoveanu, A. D., Jogl, F., Welke, P., & Thiessen, M. (2023). Extending Graph Neural Networks with Global Features. In The Second Learning on Graphs Conference (LoG 2023). The Second Learning on Graphs Conference (LoG 2023), online, Austria. OpenReview.net. https://doi.org/10.34726/5423
Downloads: PDF (365 KB) / Poster (289 KB) -
Expectation-Complete Graph Representations with Homomorphisms
/
Welke, P., Thiessen, M., Jogl, F., & Gärtner, T. (2023). Expectation-Complete Graph Representations with Homomorphisms. In A. Krause, E. Brunskill, K. Cho, B. Engelhardt, S. Sabato, & J. Scarlett (Eds.), Proceedings of the 40th International Conference on Machine Learning (pp. 36910–36925). Proceedings of Machine Learning Research.
Project: StruDL (2023–2027)
2022
-
Generalized Laplacian Positional Encoding for Graph Representation Learning
/
Maskey, S., Parviz, A., Thiessen, M., Stärk, H., Sadikaj, Y., & Maron, H. (2022, December 3). Generalized Laplacian Positional Encoding for Graph Representation Learning [Poster Presentation]. NeurIPS 2022 Workshop on Symmetry and Geometry in Neural Representations, New Orleans, United States of America (the). https://doi.org/10.34726/3908
Download: Paper (1.74 MB) -
Expectation Complete Graph Representations Using Graph Homomorphisms
/
Welke, P., Thiessen, M., & Gärtner, T. (2022, November 30). Expectation Complete Graph Representations Using Graph Homomorphisms [Poster Presentation]. First Learning on Graphs Conference (LoG 2022), Unknown. https://doi.org/10.34726/3883
Download: Accepted Paper (294 KB) - LieGG: Studying Learned Lie Group Generators / Moskalev, A., Sepliarskaia, A., Sosnovik, I., & Smeulders, A. (2022). LieGG: Studying Learned Lie Group Generators. In Advances in Neural Information Processing Systems 35 (NeurIPS 2022). Advances in Neural Information Processing Systems 35 (NeurIPS 2022), New Orleans, United States of America (the). http://hdl.handle.net/20.500.12708/175981
-
Active Learning of Classifiers with Label and Seed Queries
/
Bressan, M., Cesa-Bianchi, N., Lattanzi, S., Paudice, A., & Thiessen, M. (2022). Active Learning of Classifiers with Label and Seed Queries. In Advances in Neural Information Processing Systems 35 (NeurIPS 2022). Thirty-Sixth Conference on Neural Information Processing Systems (NeurIPS 2022), New Orleans, Louisiana, United States of America (the). Neural information processing systems foundation. https://doi.org/10.34726/4021
Downloads: Full paper (387 KB) / Supplemantary material (294 KB) -
Expectation Complete Graph Representations using Graph Homomorphisms
/
Thiessen, M., Pascal Welke, & Gärtner, T. (2022, October 25). Expectation Complete Graph Representations using Graph Homomorphisms [Presentation]. Workshop: Hot Topics in Graph Neural Networks, Kassel, Germany. http://hdl.handle.net/20.500.12708/135860
Download: slides of invited talk (1.26 MB) -
Expectation Complete Graph Representations Using Graph Homomorphisms
/
Thiessen, M., Welke, P., & Gärtner, T. (2022, October 21). Expectation Complete Graph Representations Using Graph Homomorphisms [Poster Presentation]. New Frontiers in Graph Learning (GLFrontiers) NeurIPS 2022 Workshop, New Orleans, United States of America (the). https://doi.org/10.34726/3863
Download: Full paper (304 KB) -
Weisfeiler and Leman Return with Graph Transformations
/
Jogl, F., Thiessen, M., & Gärtner, T. (2022). Weisfeiler and Leman Return with Graph Transformations. In 18th International Workshop on Mining and Learning with Graphs - Accepted Papers. 18th International Workshop on Mining and Learning with Graphs, Grenoble, France. https://doi.org/10.34726/3829
Download: Full paper as PDF (439 KB) - Unlearning Protected User Attributes in Recommendations with Adversarial Training / Ganhör, C., Penz, D., Rekabsaz, N., Lesota, O., & Schedl, M. (2022). Unlearning Protected User Attributes in Recommendations with Adversarial Training. In SIGIR ’22: Proceedings of the 45th International ACM SIGIR Conference on Research and Development in Information Retrieval (pp. 2142–2147). https://doi.org/10.1145/3477495.3531820
- EmoMTB: Emotion-aware Music Tower Blocks / Melchiorre, A. B., Penz, D., Ganhör, C., Lesota, O., Fragoso, V., Friztl, F., Parada-Cabaleiro, E., Schubert, F., & Schedl, M. (2022). EmoMTB: Emotion-aware Music Tower Blocks. In ICMR ’22: Proceedings of the 2022 International Conference on Multimedia Retrieval (pp. 206–210). https://doi.org/10.1145/3512527.3531351
-
Reducing Learning on Cell Complexes to Graphs
/
Jogl, F., Thiessen, M., & Gärtner, T. (2022). Reducing Learning on Cell Complexes to Graphs. In ICLR 2022 Workshop on Geometrical and Topological Representation Learning. ICLR 2022 Workshop on Geometrical and Topological Representation Learning, Unknown. https://doi.org/10.34726/3421
Download: Paper as PDF (263 KB) -
Dojo: A Benchmark for Large Scale Multi-Task Reinforcement Learning
/
Schmidt, D. (2022). Dojo: A Benchmark for Large Scale Multi-Task Reinforcement Learning. In ALOE 2022. Accepted Papers. Workshop on Agent Learning in Open-Endedness (ALOE) at ICLR 2022, Unknown. https://doi.org/10.34726/4263
Download: PDF (5 MB) - LFM-2b: A Dataset of Enriched Music Listening Events for Recommender Systems Research and Fairness Analysis / Schedl, M., Brandl, S., Lesota, O., Parada-Cabaleiro, E., Penz, D., & Rekabsaz, N. (2022). LFM-2b: A Dataset of Enriched Music Listening Events for Recommender Systems Research and Fairness Analysis. In ACM SIGIR Conference on Human Information Interaction and Retrieval. ACM. https://doi.org/10.1145/3498366.3505791
- Kernel Methods for Predicting Yields of Chemical Reactions / Haywood, A. L., Redshaw, J., Hanson-Heine, M. W. D., Taylor, A., Brown, A., Mason, A. M., Gärtner, T., & Hirst, J. D. (2022). Kernel Methods for Predicting Yields of Chemical Reactions. Journal of Chemical Information and Modeling, 62(9), 2077–2092. https://doi.org/10.1021/acs.jcim.1c00699
- One-Shot Learning of Ensembles of Temporal Logic Formulas for Anomaly Detection in Cyber-Physical Systems / Indri, P., Bartoli, A., Medvet, E., & Nenzi, L. (2022). One-Shot Learning of Ensembles of Temporal Logic Formulas for Anomaly Detection in Cyber-Physical Systems. In EuroGP 2022: Genetic Programming (pp. 34–50). Springer-Verlag. https://doi.org/10.1007/978-3-031-02056-8_3
- Online learning of convex sets on graphs / Thiessen, M., & Gärtner, T. (2022). Online learning of convex sets on graphs. In Joint European Conference on Machine Learning and Knowledge Discovery in Databases. Joint European Conference on Machine Learning and Knowledge Discovery in Databases (ECML PKDD 2022), Grenoble, France.
2021
-
Fast and Data-Efficient Training of Rainbow: an Experimental Study on Atari
/
Schmidt, D., & Schmied, T. (2021, December 13). Fast and Data-Efficient Training of Rainbow: an Experimental Study on Atari [Poster Presentation]. Deep RL Workshop NeurIPS 2021, Online, Unknown. https://doi.org/10.34726/3909
Download: Presentation Paper (5.87 MB) -
Active Learning Convex Halfspaces on Graphs
/
Thiessen, M., & Gärtner, T. (2021). Active Learning Convex Halfspaces on Graphs. In SubSetML @ ICML2021: Subset Selection in Machine Learning: From Theory to Practice. SubSetML: Subset Selection in Machine Learning: From Theory to Practice, Unknown. https://doi.org/10.34726/3901
Download: Accepted full paper with appendix (2.27 MB) - Team JKU-AIWarriors in the ACM Recommender Systems Challenge 2021: Lightweight XGBoost Recommendation Approach Leveraging User Features / Krauck, A., Penz, D., & Schedl, M. (2021). Team JKU-AIWarriors in the ACM Recommender Systems Challenge 2021: Lightweight XGBoost Recommendation Approach Leveraging User Features. In RecSysChallenge ’21: Proceedings of the Recommender Systems Challenge 2021. ACM. https://doi.org/10.1145/3487572.3487874
-
Active Learning of Convex Halfspaces on Graphs
/
Thiessen, M., & Gärtner, T. (2021). Active Learning of Convex Halfspaces on Graphs. In Advances in Neural Information Processing Systems 34 (NeurIPS 2021) (pp. 1–13). https://doi.org/10.34726/1841
Download: PDF (1.06 MB) - Active Learning of Convex Halfspaces on Graphs / Thiessen, M., & Gärtner, T. (2021). Active Learning of Convex Halfspaces on Graphs. In Advances in Neural Information Processing Systems 34. Advances in Neural Information Processing Systems 34. http://hdl.handle.net/20.500.12708/58787
2020
-
Efficient Reinforcement Learning via Self-supervised learning and Model-based methods
/
Schmied, T., & Thiessen, M. (2020). Efficient Reinforcement Learning via Self-supervised learning and Model-based methods. In Challenges of Real-World RL. NeurIPS 2020 Workshop. Accepted Papers. 34th Conference on Neural Information Processing Systems (NeurIPS 2020), Vancouver, Canada. https://doi.org/10.34726/4524
Download: Accepted paper (236 KB) -
Active Learning on Graphs with Geodesically Convex Classes
/
Thiessen, M., & Gärtner, T. (2020). Active Learning on Graphs with Geodesically Convex Classes. In Proceedings of 16th International Workshop on Mining and Learning with Graphs (MLG’20). 16th International Workshop on Mining and Learning with Graphs, Austria. https://doi.org/10.34726/3467
Download: author's original (729 KB) - Machine Learning for Chemical Synthesis / Haywood, A. L., Redshaw, J., Gärtner, T., Taylor, A., Mason, A. M., & Hirst, J. D. (2020). Machine Learning for Chemical Synthesis. In H. M. Cartwright (Ed.), Machine Learning in Chemistry : The Impact of Artificial Intelligence (pp. 169–194). The Royal Society of Chemistry. https://doi.org/10.1039/9781839160233-00169
2019
- Bayesian estimation of physical and geometrical parameters for nanocapacitor array biosensors / Stadlbauer, B., Cossettini, A., Morales Escalante, J. A., Pasterk, D., Scarbolo, P., Taghizadeh, L., Heitzinger, C., & Selmi, L. (2019). Bayesian estimation of physical and geometrical parameters for nanocapacitor array biosensors. Journal of Computational Physics, 397, Article 108874. https://doi.org/10.1016/j.jcp.2019.108874
Theses
-
Adaptive Foreign Exchange Hedging Strategies Using Deep Reinforcement Learning
/
Raguz, B. (2025). Adaptive Foreign Exchange Hedging Strategies Using Deep Reinforcement Learning [Diploma Thesis, Technische Universität Wien]. reposiTUm. https://doi.org/10.34726/hss.2025.121287
Download: PDF (890 KB) -
Operational Data-Driven SoH Estimation and Prediction for Maritime Battery Systems
/
Komic, N. (2025). Operational Data-Driven SoH Estimation and Prediction for Maritime Battery Systems [Diploma Thesis, Technische Universität Wien]. reposiTUm. https://doi.org/10.34726/hss.2025.131831
Download: PDF (2.44 MB) -
Graph Representations and Neural Network Architectures for Reaction Barrier Height Prediction
/
De Landsheere, J. (2025). Graph Representations and Neural Network Architectures for Reaction Barrier Height Prediction [Diploma Thesis, Technische Universität Wien]. reposiTUm. https://doi.org/10.34726/hss.2025.128222
Download: PDF (1.24 MB) -
Reliability in Reinforcement Learning and Off-Policy Evaluation
/
aus der Schmitten, J. (2025). Reliability in Reinforcement Learning and Off-Policy Evaluation [Diploma Thesis, Technische Universität Wien]. reposiTUm. https://doi.org/10.34726/hss.2025.116800
Download: PDF (1.24 MB) -
Probabilistic Verification of Black-Box Systems
/
Blohm, P. (2025). Probabilistic Verification of Black-Box Systems [Diploma Thesis, Technische Universität Wien]. reposiTUm. https://doi.org/10.34726/hss.2025.128785
Download: PDF (14.8 MB) - Modelling and adaptive control of industrial waste wood and residue boilers using neural networks / Voith, S. (2025). Modelling and adaptive control of industrial waste wood and residue boilers using neural networks [Diploma Thesis, Technische Universität Wien]. reposiTUm. https://doi.org/10.34726/hss.2025.124592
-
Time Series Prediction with Attention-Based Transformer Models
/
Kund-Leitner, A. (2025). Time Series Prediction with Attention-Based Transformer Models [Diploma Thesis, Technische Universität Wien]. reposiTUm. https://doi.org/10.34726/hss.2025.114552
Download: PDF (2 MB) -
Transforming Text into Motion: Fundamentals of Diffusion Transformers for Text-to-Video Generation
/
Halbeisen, C. (2025). Transforming Text into Motion: Fundamentals of Diffusion Transformers for Text-to-Video Generation [Diploma Thesis, Technische Universität Wien]. reposiTUm. https://doi.org/10.34726/hss.2025.126680
Download: PDF (2.06 MB) -
Evaluating Reinforcement-Learning-based Sepsis Treatments via Tabular and Continuous Stationary Distribution Correction Estimation
/
Weiss, R. (2025). Evaluating Reinforcement-Learning-based Sepsis Treatments via Tabular and Continuous Stationary Distribution Correction Estimation [Diploma Thesis, Technische Universität Wien]. reposiTUm. https://doi.org/10.34726/hss.2025.126219
Download: PDF (4.72 MB) -
Applications of concentration inequalities in distributional reinforcement learning
/
Mayer, F. (2024). Applications of concentration inequalities in distributional reinforcement learning [Diploma Thesis, Technische Universität Wien]. reposiTUm. https://doi.org/10.34726/hss.2025.124987
Download: PDF (785 KB) -
One-class domain adaptation via meta-learning
/
Holly, S. (2024). One-class domain adaptation via meta-learning [Diploma Thesis, Technische Universität Wien]. reposiTUm. https://doi.org/10.34726/hss.2024.125980
Download: PDF (1.13 MB) -
Antibody-antigen binding affinity prediction through the use of geometric deep learning : a framework for binding affinity prediction with graph neural networks
/
Traxler, F. (2023). Antibody-antigen binding affinity prediction through the use of geometric deep learning : a framework for binding affinity prediction with graph neural networks [Diploma Thesis, Technische Universität Wien]. reposiTUm. https://doi.org/10.34726/hss.2023.105645
Download: PDF (4.87 MB) -
On SGD with momentum
/
Plattner, M. (2023). On SGD with momentum [Diploma Thesis, Technische Universität Wien]. reposiTUm. https://doi.org/10.34726/hss.2023.106165
Download: PDF (1.69 MB) -
Do we need to Improve message passing? Improving graph neural networks with graph transformations
/
Jogl, F. (2022). Do we need to Improve message passing? Improving graph neural networks with graph transformations [Diploma Thesis, Technische Universität Wien]. reposiTUm. https://doi.org/10.34726/hss.2022.103141
Download: PDF (897 KB) -
Self-supervision, data augmentation and online fine-tuning for offline RL
/
Schmied, T. (2022). Self-supervision, data augmentation and online fine-tuning for offline RL [Diploma Thesis, Technische Universität Wien]. reposiTUm. https://doi.org/10.34726/hss.2022.89725
Download: PDF (4.85 MB) -
Representation learning for variable-sized multiple sequence alignments
/
Drucks, T. (2021). Representation learning for variable-sized multiple sequence alignments [Diploma Thesis, Technische Universität Wien]. reposiTUm. https://doi.org/10.34726/hss.2021.88083
Download: PDF (24.3 MB) -
Recommending reviewers for theses using artificial intelligence
/
Penz, D. (2021). Recommending reviewers for theses using artificial intelligence [Diploma Thesis, Technische Universität Wien]. reposiTUm. https://doi.org/10.34726/hss.2021.76463
Download: PDF (2.07 MB)
Awards
-
Clemens Heitzinger:
PDE Models for Nanotechnology
2013 / START Prize / Austria / Website -
Clemens Heitzinger:
START-Preis
2013 / START-Programm / Austria -
Clemens Heitzinger:
Erwin Schrödinger Fellowship (FWF)
2003 / Schrödinger-Stipendium / Austria
And more…
Soon, this page will include additional information such as reference projects, conferences, events, and other research activities.
Until then, please visit Machine Learning’s research profile in TISS .