Artificial Intelligence Techniques E192-07
Our research aims at enabling machines to mimic human-like intelligence, via models and algorithms to process vast amounts of data, recognize patterns, and make decisions, using techniques from machine and deep learning as well as symbolic reasoning.
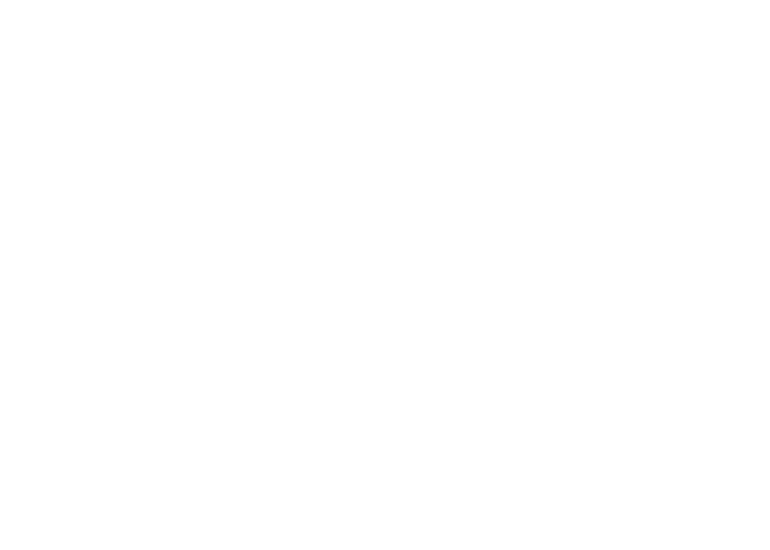
Contact
- Head: Thomas Lukasiewicz
- Web: informatics.tuwien.ac.at/orgs/e192-07
- Location: Erzherzog-Johann-Platz 1
On This Page
About
Our research aims at enabling machines to mimic human-like intelligence, via models and algorithms to process vast amounts of data, recognize patterns, and make decisions, using techniques from machine and deep learning as well as symbolic reasoning.
In our research activities, we cover all modalities, including natural language processing and computer vision. We focus especially on (i) explainable AI, (ii) deep learning with logical constraints for safe AI , (iii) hybrid model-based approaches to explainable, fair, and robust AI, (iv) general AI via predictive coding and active inference, and (v) intelligent applications, such as in healthcare and law.
The research Unit Artificial Intelligence Techniques is part of the Institute of Logic and Computation.
Professors
Scientific Staff
Visiting Researchers
Administrative Staff
Courses
2024W
- Bachelor Thesis for Computer Science and Business Informatics / 192.143 / PR
- Deep Learning for Natural Language Processing / 192.039 / VU
- Project in Computer Science 1 / 192.021 / PR
- Project in Computer Science 2 / 192.022 / PR
- Scientific Research and Writing / 193.052 / SE
- Seminar for Master Students in Logic and Computation / 180.773 / SE
- Seminar for PhD Students / 192.031 / SE
- Seminar in Artificial Intelligence : Neuroscience-based Artificial Intelligence / 192.047 / SE
- Seminar in Knowledge Representation and Reasoning : Neurosymbolic Artificial Intelligence / 192.048 / SE
2025S
- Bachelor Thesis for Computer Science and Business Informatics / 192.143 / PR
- Deep Learning for Natural Language Processing / 192.039 / VU
- Introduction to Deep Learning / 192.151 / VU
- Project in Computer Science 1 / 192.021 / PR
- Project in Computer Science 2 / 192.022 / PR
- Scientific Research and Writing / 193.052 / SE
- Seminar for Master Students in Logic and Computation / 180.773 / SE
- Seminar for PhD Students / 192.031 / SE
- Seminar in Artificial Intelligence : Neuroscience-based Artificial Intelligence / 192.047 / SE
- Seminar in Knowledge Representation and Reasoning : Neurosymbolic Artificial Intelligence / 192.048 / SE
Projects
-
Explainable Artificial Intelligence in Healthcare
2023 – 2027 / AXA
Publications
2025
- Aggregated Mutual Learning between CNN and Transformer for semi-supervised medical image segmentation / Xu, Z., Wang, H., Yang, R., Yang, Y., Liu, W., & Lukasiewicz, T. (2025). Aggregated Mutual Learning between CNN and Transformer for semi-supervised medical image segmentation. Knowledge-Based Systems, 311, Article 113005. https://doi.org/10.1016/j.knosys.2025.113005
- Hybrid Reinforced Medical Report Generation With M-Linear Attention and Repetition Penalty / Xu, Z., Xu, W., Wang, R., Chen, J., Qi, C., & Lukasiewicz, T. (2025). Hybrid Reinforced Medical Report Generation With M-Linear Attention and Repetition Penalty. IEEE Transactions on Neural Networks and Learning Systems, 36(2), 2206–2220. https://doi.org/10.1109/TNNLS.2023.3343391
- Self-Supervised Medical Image Segmentation Using Deep Reinforced Adaptive Masking / Xu, Z., Liu, Y., Xu, G., & Lukasiewicz, T. (2025). Self-Supervised Medical Image Segmentation Using Deep Reinforced Adaptive Masking. IEEE Transactions on Medical Imaging, 44(1), 180–193. https://doi.org/10.1109/TMI.2024.3436608
2024
- Fool Me Once? Contrasting Textual and Visual Explanations in a Clinical Decision-Support Setting / Kayser, M., Menzat, B. I., Emde, C., Bercean, B., Novak, A., Espinosa, A., Papiez, B. W., Gaube, S., Lukasiewicz, T., & Camburu, O.-M. (2024). Fool Me Once? Contrasting Textual and Visual Explanations in a Clinical Decision-Support Setting. In Proceedings of the 2024 Conference on Empirical Methods in Natural Language Processing (pp. 18891–18919). Association for Computational Linguistics. https://doi.org/10.18653/v1/2024.emnlp-main.1051
- CCN⁺: A Neuro-symbolic Framework for Deep Learning with Requirements / Giunchiglia, E., Tatomir, A., Stoian, M. C., & Lukasiewicz, T. (2024). CCN+: A Neuro-symbolic Framework for Deep Learning with Requirements . International Journal of Approximate Reasoning, 171, Article 109124. https://doi.org/10.1016/j.ijar.2024.109124
- Correcting Flaws in Common Disentanglement Metrics / Mahon, L., Sha, L., & Lukasiewicz, T. (2024). Correcting Flaws in Common Disentanglement Metrics. Transactions on Machine Learning Research, 2024.
- Evaluating Language Models for Mathematics through Interactions / Collins, K. M., Jiang, A. Q., Frieder, S., Wong, L., Zilka, M., Bhatt, U., Lukasiewicz, T., Wu, Y., Tenenbaum, J. B., Hart, W., Gowers, T., Li, W., Weller, A., & Jamnik, M. (2024). Evaluating Language Models for Mathematics through Interactions. Proceedings of the National Academy of Sciences of the United States of America, 121(24), Article e2318124121. https://doi.org/10.1073/pnas.2318124121
- Pre-training and diagnosing knowledge base completion models / Kocijan, V., Jang, M., & Lukasiewicz, T. (2024). Pre-training and diagnosing knowledge base completion models. Artificial Intelligence, 329, Article 104081. https://doi.org/10.1016/j.artint.2024.104081
- Hard Regularization to Prevent Deep Online Clustering Collapse without Data Augmentation / Mahon, L., & Lukasiewicz, T. (2024). Hard Regularization to Prevent Deep Online Clustering Collapse without Data Augmentation. In Proceedings of the 38th AAAI Conference on Artificial Intelligence : AAAI-24 Technical Tracks 13 (pp. 14281–14288). AAAI Press. https://doi.org/10.1609/aaai.v38i13.29340
- Automatic data augmentation for medical image segmentation using Adaptive Sequence-length based Deep Reinforcement Learning / Xu, Z., Wang, S., Xu, G., Liu, Y., Yu, M., Zhang, H., Lukasiewicz, T., & Gu, J. (2024). Automatic data augmentation for medical image segmentation using Adaptive Sequence-length based Deep Reinforcement Learning. Computers in Biology and Medicine, 169, Article 107877. https://doi.org/10.1016/J.COMPBIOMED.2023.107877
-
Minimum description length clustering to measure meaningful image complexity
/
Mahon, L., & Lukasiewicz, T. (2024). Minimum description length clustering to measure meaningful image complexity. Pattern Recognition, 145, Article 109889. https://doi.org/10.1016/j.patcog.2023.109889
Download: Article (3.29 MB) - PhaCIA-TCNs: Short-Term Load Forecasting Using Temporal Convolutional Networks With Parallel Hybrid Activated Convolution and Input Attention / Xu, Z., Yu, Z., Zhang, H., Chen, J., Gu, J., Lukasiewicz, T., & Leung, V. C. M. (2024). PhaCIA-TCNs: Short-Term Load Forecasting Using Temporal Convolutional Networks With Parallel Hybrid Activated Convolution and Input Attention. IEEE Transactions on Network Science and Engineering, 11(1), 427–438. https://doi.org/10.1109/TNSE.2023.3300744
- A Stable, Fast, and Fully Automatic Learning Algorithm for Predictive Coding Networks / Tommaso Salvatori, Song, Y., Yordanov, Y., Millidge, B., Sha, L., Emde, C., Xu, Z., Bogacz, R., & Thomas Lukasiewicz. (2024). A Stable, Fast, and Fully Automatic Learning Algorithm for Predictive Coding Networks. In The Twelfth International Conference on Learning Representations (p. 25). http://hdl.handle.net/20.500.12708/211106
- Multi-ConDoS: Multimodal Contrastive Domain Sharing Generative Adversarial Networks for Self-Supervised Medical Image Segmentation / Zhang, J., Zhang, S., Shen, X., Lukasiewicz, T., & Xu, Z. (2024). Multi-ConDoS: Multimodal Contrastive Domain Sharing Generative Adversarial Networks for Self-Supervised Medical Image Segmentation. IEEE Transactions on Medical Imaging, 43(1), 76–95. https://doi.org/10.1109/TMI.2023.3290356
- Text attribute control via closed-loop disentanglement / Sha, L., & Thomas Lukasiewicz. (2024). Text attribute control via closed-loop disentanglement. Transactions of the Association for Computational Linguistics, 12. https://doi.org/10.1162/tacl_a_00640
- The IMO Small Challenge: Not-Too-Hard Olympiad Math Datasets for LLMs / Frieder, S., Olšák, M., Berner, J., & Lukasiewicz, T. (2024). The IMO Small Challenge: Not-Too-Hard Olympiad Math Datasets for LLMs. In The Second Tiny Papers Track at ICLR 2024. The Twelfth International Conference on Learning Representations (ICLR 2024), Wien, Austria. http://hdl.handle.net/20.500.12708/210292
- How Realistic Is Your Synthetic Data? Constraining Deep Generative Models for Tabular Data / Stoian, M. C., Dyrmishi, S., Cordy, M., Lukasiewicz, T., & Giunchiglia, E. (2024). How Realistic Is Your Synthetic Data? Constraining Deep Generative Models for Tabular Data. In The Twelfth International Conference on Learning Representations. 12th International Conference on Learning Representations (ICLR 2024), Wien, Austria. http://hdl.handle.net/20.500.12708/210296
- Predictive Coding beyond Correlations / Salvatori, T., Pinchetti, L., M’Charrak, A., Millidge, B., & Lukasiewicz, T. (2024). Predictive Coding beyond Correlations. In Forty-first International Conference on Machine Learning. Forty-first International Conference on Machine Learning, ICML 2024, Wien, Austria. http://hdl.handle.net/20.500.12708/210295
- PiShield: A NeSy Framework for Learning with Requirements / Stoian, M. C., Tatomir, A., Lukasiewicz, T., & Giunchiglia, E. (2024). PiShield: A NeSy Framework for Learning with Requirements. In K. Larson (Ed.), IJCAI ’24: Proceedings of the Thirty-Third International Joint Conference on Artificial Intelligence (pp. 8805–8809). Association for Computing Machinery. https://doi.org/10.24963/ijcai.2024/1037
-
Inferring neural activity before plasticity as a foundation for learning beyond backpropagation
/
Song, Y., Millidge, B., Salvatori, T., Lukasiewicz, T., Xu, Z., & Bogacz, R. (2024). Inferring neural activity before plasticity as a foundation for learning beyond backpropagation. Nature Neuroscience. https://doi.org/10.1038/s41593-023-01514-1
Download: Article (6.58 MB) - Bad Predictive Coding Activation Functions / Frieder, S., Pinchetti, L., & Lukasiewicz, T. (2024). Bad Predictive Coding Activation Functions. In The Second Tiny Papers Track at ICLR 2024. The Twelfth International Conference on Learning Representations (ICLR 2024), Wien, Austria.
- Cross-domain attention-guided generative data augmentation for medical image analysis with limited data / Xu, Z., Tang, J., Qi, C., Yao, D., Liu, C., Zhan, Y., & Lukasiewicz, T. (2024). Cross-domain attention-guided generative data augmentation for medical image analysis with limited data. Computers in Biology and Medicine, 168, Article 107744. https://doi.org/10.1016/J.COMPBIOMED.2023.107744
2023
- Large Language Models for Mathematicians / Frieder, S., Berner, J., Petersen, P., & Lukasiewicz, T. (2023). Large Language Models for Mathematicians. Internationale Mathematische Nachrichten, 254, 1–20. http://hdl.handle.net/20.500.12708/192474
- RIRGAN: An end-to-end lightweight multi-task learning method for brain MRI super-resolution and denoising / Yu, M., Guo, M., Zhang, S., Zhan, Y., Zhao, M., Lukasiewicz, T., & Xu, Z. (2023). RIRGAN: An end-to-end lightweight multi-task learning method for brain MRI super-resolution and denoising. Computers in Biology and Medicine, 167, Article 107632. https://doi.org/10.1016/j.compbiomed.2023.107632
- The Defeat of the Winograd Schema Challenge / Kocijan, V., Davis, E., Lukasiewicz, T., Marcus, G., & Morgenstern, L. (2023). The Defeat of the Winograd Schema Challenge. Artificial Intelligence, 325, Article 103971. https://doi.org/10.1016/j.artint.2023.103971
- Collaborative Attention Guided Multi-Scale Feature Fusion Network for Medical Image Segmentation / Xu, Z., Tian, B., Liu, S., Wang, X., Yuan, D., Gu, J., Chen, J., Lukasiewicz, T., & Leung, V. C. M. (2023). Collaborative Attention Guided Multi-Scale Feature Fusion Network for Medical Image Segmentation. IEEE Transactions on Network Science and Engineering, 1–15. https://doi.org/10.1109/TNSE.2023.3332810
- EFPN: Effective medical image detection using feature pyramid fusion enhancement / Xu, Z., Zhang, X., Zhang, H., Liu, Y., Zhan, Y., & Lukasiewicz, T. (2023). EFPN: Effective medical image detection using feature pyramid fusion enhancement. Computers in Biology and Medicine, 163, Article 107149. https://doi.org/https://doi.org/10.1016/j.compbiomed.2023.107149
- ROAD-R: the autonomous driving dataset with logical requirements / Giunchiglia, E., Stoian, M. C., Khan, S., Cuzzolin, F., & Lukasiewicz, T. (2023). ROAD-R: the autonomous driving dataset with logical requirements. Machine Learning, 112, 3261–3291. https://doi.org/10.1007/s10994-023-06322-z
- μ-Net: Medical image segmentation using efficient and effective deep supervision / Yuan, D., Xu, Z., Tian, B., Wang, H., Zhan, Y., & Lukasiewicz, T. (2023). μ-Net: Medical image segmentation using efficient and effective deep supervision. Computers in Biology and Medicine, 160, Article 106963. https://doi.org/10.1016/j.compbiomed.2023.106963
- Recurrent predictive coding models for associative memory employing covariance learning / Tang, M., Salvatori, T., Millidge, B., Song, Y., Lukasiewicz, T., & Bogacz, R. (2023). Recurrent predictive coding models for associative memory employing covariance learning. PLoS Computational Biology, 19(4), e1010719. https://doi.org/10.1371/journal.pcbi.1010719
- PAC-Net: Multi-pathway FPN with position attention guided connections and vertex distance IoU for 3D medical image detection / Xu, Z., Li, T., Liu, Y., Zhan, Y., Chen, J., & Lukasiewicz, T. (2023). PAC-Net: Multi-pathway FPN with position attention guided connections and vertex distance IoU for 3D medical image detection. Frontiers in Bioengineering and Biotechnology, 11, Article 1049555. https://doi.org/10.3389/fbioe.2023.1049555
- Painless and accurate medical image analysis using deep reinforcement learning with task-oriented homogenized automatic pre-processing / Yuan, D., Liu, Y., Xu, Z., Zhan, Y., Chen, J., & Lukasiewicz, T. (2023). Painless and accurate medical image analysis using deep reinforcement learning with task-oriented homogenized automatic pre-processing. Computers in Biology and Medicine, 153, Article 106487. https://doi.org/10.1016/j.compbiomed.2022.106487
- Rationalizing predictions by adversarial information calibration / Sha, L., Camburu, O.-M., & Lukasiewicz, T. (2023). Rationalizing predictions by adversarial information calibration. Artificial Intelligence, 315, 103828. https://doi.org/https://doi.org/10.1016/j.artint.2022.103828
- Hi-BEHRT: Hierarchical Transformer-Based Model for Accurate Prediction of Clinical Events Using Multimodal Longitudinal Electronic Health Records / Li, Y., Mamouei, M., Salimi-Khorshidi, G., Rao, S., Hassaine, A., Canoy, D., Lukasiewicz, T., & Rahimi, K. (2023). Hi-BEHRT: Hierarchical Transformer-Based Model for Accurate Prediction of Clinical Events Using Multimodal Longitudinal Electronic Health Records. IEEE Journal of Biomedical and Health Informatics, 27(2), 1106–1117. https://doi.org/10.1109/JBHI.2022.3224727
- Consistency Analysis of ChatGPT / Jang, M., & Lukasiewicz, T. (2023). Consistency Analysis of ChatGPT. In H. Bouamor, J. Pino, & K. Bali (Eds.), Proceedings of the 2023 Conference on Empirical Methods in Natural Language Processing (pp. 15970–15985). Association for Computational Linguistics. https://doi.org/10.18653/v1/2023.emnlp-main.991
- Mathematical Capabilities of ChatGPT / Frieder, S., Pinchetti, L., Chevalier, A., Griffiths, R.-R., Salvatori, T., Lukasiewicz, T., Petersen, P., & Berner, J. (2023). Mathematical Capabilities of ChatGPT. In Advances in Neural Information Processing Systems 36 pre-proceedings (NeurIPS 2023). 37th Conference on Neural Information Processing Systems (NeurIPS 2023), New Orleans, United States of America (the). http://hdl.handle.net/20.500.12708/194133
- MvCo-DoT: Multi-View Contrastive Domain Transfer Network for Medical Report Generation / Wang, R., Wang, X., Xu, Z., Xu, W., Chen, J., & Lukasiewicz, T. (2023). MvCo-DoT: Multi-View Contrastive Domain Transfer Network for Medical Report Generation. In ICASSP 2023 - 2023 IEEE International Conference on Acoustics, Speech and Signal Processing (ICASSP). 2023 International Conference on Acoustics, Speech, and Signal Processing, Rhodes, Greece. IEEE. https://doi.org/10.1109/ICASSP49357.2023.10095254
- MPS-AMS: Masked Patches Selection and Adaptive Masking Strategy Based Self-Supervised Medical Image Segmentation / Wang, X., Wang, R., Tian, B., Zhang, J., Zhang, S., Chen, J., Lukasiewicz, T., & Xu, Z. (2023). MPS-AMS: Masked Patches Selection and Adaptive Masking Strategy Based Self-Supervised Medical Image Segmentation. In ICASSP 2023 - 2023 IEEE International Conference on Acoustics, Speech and Signal Processing (ICASSP). 2023 IEEE International Conference on Acoustics, Speech and Signal Processing, Rhodes, Greece. IEEE. https://doi.org/10.1109/ICASSP49357.2023.10094657
- Associative Memories in the Feature Space / Salvatori, T., Millidge, B., Song, Y., Bogacz, R., & Lukasiewicz, T. (2023). Associative Memories in the Feature Space. In K. Gal, A. Nowé, & G. J. Nalepa (Eds.), 26th European Conference on Artificial Intelligence, September 30–October 4, 2023, Kraków, Poland – Including 12th Conference on Prestigious Applications of Intelligent Systems (PAIS 2023) (pp. 2065–2072). IOS Press. https://doi.org/10.3233/FAIA230500
- Multi-Head Feature Pyramid Networks for Breast Mass Detection / Zhang, H., Xu, Z., Yao, D., Zhang, S., Chen, J., & Thomas Lukasiewicz. (2023). Multi-Head Feature Pyramid Networks for Breast Mass Detection. In ICASSP 2023 - 2023 IEEE International Conference on Acoustics, Speech and Signal Processing (ICASSP). 2023 IEEE International Conference on Acoustics, Speech and Signal Processing, Rhodes, Greece. IEEE. https://doi.org/10.1109/ICASSP49357.2023.10095967
- Adaptive-Masking Policy with Deep Reinforcement Learning for Self-Supervised Medical Image Segmentation / Xu, G., Wang, S., Lukasiewicz, T., & Xu, Z. (2023). Adaptive-Masking Policy with Deep Reinforcement Learning for Self-Supervised Medical Image Segmentation. In 2023 IEEE International Conference on Multimedia and Expo (ICME) (pp. 2285–2290). IEEE. https://doi.org/10.1109/ICME55011.2023.00390
- NP-SemiSeg: When Neural Processes meet Semi-Supervised Semantic Segmentation / Wang, J., Massiceti, D., Hu, X., Pavlovic, V., & Lukasiewicz, T. (2023). NP-SemiSeg: When Neural Processes meet Semi-Supervised Semantic Segmentation. In A. Krause, E. Brunskill, K. Cho, B. Engelhardt, S. Sabato, & J. Scarlett (Eds.), PMLR Proceedings of Machine Learning Research. http://hdl.handle.net/20.500.12708/192515
- KNOW How to Make Up Your Mind! Adversarially Detecting and Remedying Inconsistencies in Natural Language Explanations / Jang, M., Majumder, B. P., McAuley, J., Lukasiewicz, T., & Camburu, O.-M. (2023). KNOW How to Make Up Your Mind! Adversarially Detecting and Remedying Inconsistencies in Natural Language Explanations. In A. Rogers, J. Boyd-Graber, & N. Okazaki (Eds.), Proceedings of the 61st Annual Meeting of the Association for Computational Linguistics (Volume 2: Short Papers) (pp. 540–553). Association for Computational Linguistics. https://doi.org/10.18653/v1/2023.acl-short.47
- Exploiting T-norms for Deep Learning in Autonomous Driving / Stoian, M. C., Giunchiglia, E., & Lukasiewicz, T. (2023). Exploiting T-norms for Deep Learning in Autonomous Driving. In A. S. d’Avila Garcez, T. R. Besold, M. Gori, & E. Jimenez-Ruiz (Eds.), Proceedings of the 17th International Workshop on Neural-Symbolic Learning and Reasoning (NeSy 2023) (pp. 369–380). http://hdl.handle.net/20.500.12708/193631
- Faithfulness Tests for Natural Language Explanations / Atanasova, P., Camburu, O.-M., Lioma, C., Lukasiewicz, T., Simonsen, J. G., & Augenstein, I. (2023). Faithfulness Tests for Natural Language Explanations. In Association for Computational Linguistics (Ed.), Proceedings of the 61st Annual Meeting of the Association for Computational Linguistics (Volume 2: Short Papers) (pp. 283–294). Association for Computational Linguistics. https://doi.org/10.18653/v1/2023.acl-short.25
- Complexity of Inconsistency-Tolerant Query Answering in Datalog+/- under Preferred Repairs / Lukasiewicz, T., Malizia, E., & Molinaro, C. (2023). Complexity of Inconsistency-Tolerant Query Answering in Datalog+/- under Preferred Repairs. In P. Marquis, T. C. Son, & G. Kern-Isberner (Eds.), Proceedings of 20th International Conference on Principles of Knowledge Representation and Reasoning (pp. 472–481). IJCAI Organization. https://doi.org/10.24963/kr.2023/46
- Counter−GAP: Counterfactual Bias Evaluation through Gendered Ambiguous Pronouns / Zhongbin, X., Kocijan, V., Lukasiewicz, T., & Camburu, O.-M. (2023). Counter−GAP: Counterfactual Bias Evaluation through Gendered Ambiguous Pronouns. In A. Vlachos & Isabelle Augenstein (Eds.), Proceedings of the 17th Conference of the European Chapter of the Association for Computational Linguistics (pp. 3761–3773). Association for Computational Linguistics. https://doi.org/10.18653/v1/2023.eacl-main.272
- An Empirical Analysis of Parameter-Efficient Methods for Debiasing Pre-Trained Language Models / Xie, Z., & Lukasiewicz, T. (2023). An Empirical Analysis of Parameter-Efficient Methods for Debiasing Pre-Trained Language Models. In In Proceedings of the 61st Annual Meeting of the Association for Computational Linguistics (Volume 1: Long Papers) (pp. 15730–15745). Association for Computational Linguistics. https://doi.org/10.18653/v1/2023.acl-long.876
- Efficient Deep Clustering of Human Activities and How to Improve Evaluation / Mahon, L., & Lukasiewicz, T. (2023). Efficient Deep Clustering of Human Activities and How to Improve Evaluation. In E. Khan & M. Gönen (Eds.), Proceedings of Machine Learning Research 189, 2022 (pp. 722–737). http://hdl.handle.net/20.500.12708/193628
- Improving Language Models’ Meaning Understanding and Consistency by Learning Conceptual Roles from Dictionary / Jang, M., & Lukasiewicz, T. (2023). Improving Language Models’ Meaning Understanding and Consistency by Learning Conceptual Roles from Dictionary. In H. Bouamor, J. Pino, & K. Bali (Eds.), Proceedings of the 2023 Conference on Empirical Methods in Natural Language Processing (pp. 8496–8510). Association for Computational Linguistics. https://doi.org/10.18653/v1/2023.emnlp-main.527
- Backpropagation at the Infinitesimal Inference Limit of Energy-Based Models: Unifying Predictive Coding, Equilibrium Propagation, and Contrastive Hebbian Learning / Millidge, B., Song, Y., Salvatori, T., Lukasiewicz, T., & Bogacz, R. (2023). Backpropagation at the Infinitesimal Inference Limit of Energy-Based Models: Unifying Predictive Coding, Equilibrium Propagation, and Contrastive Hebbian Learning. In The Eleventh International Conference on Learning Representations, ICLR 2023 (pp. 1–14). http://hdl.handle.net/20.500.12708/192480
- A Theoretical Framework for Inference and Learning in Predictive Coding Networks / Millidge, B., Song, Y., Salvatori, T., Lukasiewicz, T., & Bogacz, R. (2023). A Theoretical Framework for Inference and Learning in Predictive Coding Networks. In The Eleventh International Conference on Learning Representations, ICLR 2023 (pp. 1–24). http://hdl.handle.net/20.500.12708/192478
2022
- BECEL: Benchmark for Consistency Evaluation of Language Models / Jang, M., Kwon, D. S., & Lukasiewicz, T. (2022). BECEL: Benchmark for Consistency Evaluation of Language Models. In N. Calzolari, C.-R. Huang, & H. Kim (Eds.), Proceedings of the 29th International Conference on Computational Linguistics (pp. 3680–3696). International Committee on Computational Linguistics. http://hdl.handle.net/20.500.12708/192675
- Clustering Generative Adversarial Networks for Story Visualization / Li, B., Torr, P. H. S., & Lukasiewicz, T. (2022). Clustering Generative Adversarial Networks for Story Visualization. In MM ’22: Proceedings of the 30th ACM International Conference on Multimedia (pp. 769–778). Association for Computing Machinery. https://doi.org/10.1145/3503161.3548034
- Explaining Chest X-Ray Pathologies in Natural Language / Kayser, M., Emde, C., Camburu, O.-M., Parsons, G., Papiez, B., & Lukasiewicz, T. (2022). Explaining Chest X-Ray Pathologies in Natural Language. In L. Wang, Q. Dou, P. T. Fletcher, S. Speidel, & S. Li (Eds.), Medical Image Computing and Computer Assisted Intervention – MICCAI 2022 (pp. 701–713). https://doi.org/10.1007/978-3-031-16443-9_67
- NoiER: An Approach for Training more Reliable Fine-Tuned Downstream Task Models / Jang, M., & Thomas Lukasiewicz. (2022). NoiER: An Approach for Training more Reliable Fine-Tuned Downstream Task Models. IEEE/ACM Transactions on Audio, Speech and Language Processing, 30, 2514–2525. https://doi.org/10.1109/TASLP.2022.3193292
- NP-Match: When Neural Processes meet Semi-Supervised Learning / Wang, J., Lukasiewicz, T., Massiceti, D., Hu, X., Pavlovic, V., & Neophytou, A. (2022). NP-Match: When Neural Processes meet Semi-Supervised Learning. In Proceedings of the 39th International Conference on Machine Learning (pp. 22919–22934). PMLR. http://hdl.handle.net/20.500.12708/192517
- Knowledge-Grounded Self-Rationalization via Extractive and Natural Language Explanations / Majumder, B. P., Camburu, O.-M., Lukasiewicz, T., & McAuley, J. (2022). Knowledge-Grounded Self-Rationalization via Extractive and Natural Language Explanations. In K. Chaudhuri, S. Jegelka, & L. Song (Eds.), Proceedings of the 39th International Conference on Machine Learning (pp. 14786–14801). MLResearch Press. http://hdl.handle.net/20.500.12708/192473
- Explanations for Negative Query Answers under Inconsistency-Tolerant Semantics / Lukasiewicz, T., Malizia, E., & Molinaro, C. (2022). Explanations for Negative Query Answers under Inconsistency-Tolerant Semantics. In L. De Raedt (Ed.), Proceedings of the Thirty-First International Joint Conference on Artificial Intelligence (pp. 2705–2711). International Joint Conferences on Artificial Intelligence. https://doi.org/10.24963/ijcai.2022/375
- Few-Shot Out-of-Domain Transfer Learning of Natural Language Explanations in a Label-Abundant Setup / Yordanov, Y., Kocijan, V., Lukasiewicz, T., & Camburu, O.-M. (2022). Few-Shot Out-of-Domain Transfer Learning of Natural Language Explanations in a Label-Abundant Setup. In Y. Goldberg, K. Zornitsa, & Y. Zhang (Eds.), Findings of the Association for Computational Linguistics: EMNLP 2022 (pp. 3486–3501). Association for Computational Linguistics. https://doi.org/10.18653/v1/2022.findings-emnlp.255
- Image-to-Image Translation with Text Guidance / Li, B., Torr, P. H. S., & Lukasiewicz, T. (2022). Image-to-Image Translation with Text Guidance. In The 33rd British Machine Vision Conference Proceedings (pp. 1–14). http://hdl.handle.net/20.500.12708/193629
- Deep Learning with Logical Constraints / Giunchiglia, E., Stoian, M. C., & Lukasiewicz, T. (2022). Deep Learning with Logical Constraints. In L. De Raedt (Ed.), Proceedings of the Thirty-First International Joint Conference on Artificial Intelligence (pp. 5478–5485). International Joint Conferences on Artificial Intelligence. https://doi.org/10.24963/ijcai.2022/767
-
Syntactically Rich Discriminative Training: An Effective Method for Open Information Extraction
/
Mtumbuka, F., & Lukasiewicz, T. (2022). Syntactically Rich Discriminative Training: An Effective Method for Open Information Extraction. In Proceedings of the 2022 Conference on Empirical Methods in Natural Language Processing (pp. 5972–5987). Association for Computational Linguistics. https://doi.org/10.18653/v1/2022.emnlp-main.401
Download: PDF (397 KB) - Memory-Driven Text-to-Image Generation / Li, B., Torr, P. H. S., & Lukasiewicz, T. (2022). Memory-Driven Text-to-Image Generation. In The 33rd British Machine Vision Conference Proceedings. 33rd British Machine Vision Conference, London, United Kingdom of Great Britain and Northern Ireland (the). http://hdl.handle.net/20.500.12708/193654
-
Learning to Model Multimodal Semantic Alignment for Story Visualization
/
Li, B., & Lukasiewicz, T. (2022). Learning to Model Multimodal Semantic Alignment for Story Visualization. In Findings of the Association for Computational Linguistics: EMNLP 2022 (pp. 4741–4747). Association for Computational Linguistics. https://doi.org/10.18653/v1/2022.findings-emnlp.346
Download: PDF (2.68 MB) - Predictive Coding: Towards a Future of Deep Learning beyond Backpropagation? / Millidge, B., Salvatori, T., Song, Y., Bogacz, R., & Lukasiewicz, T. (2022). Predictive Coding: Towards a Future of Deep Learning beyond Backpropagation? In L. De Raedt (Ed.), Proceedings of the Thirty-First International Joint Conference on Artificial Intelligence (IJCAI-22) (pp. 5538–5545). International Joint Conferences on Artificial Intelligence. https://doi.org/10.24963/ijcai.2022/774
- Universal Hopfield Networks: A General Framework for Single-Shot Associative Memory Models / Millidge, B., Salvatori, T., Song, Y., Lukasiewicz, T., & Bogacz, R. (2022). Universal Hopfield Networks: A General Framework for Single-Shot Associative Memory Models. In Proceedings of the 39th International Conference on Machine Learning (pp. 15561–15583). http://hdl.handle.net/20.500.12708/192477
- (Non-)Convergence Results for Predictive Coding Networks / Frieder, S., & Lukasiewicz, T. (2022). (Non-)Convergence Results for Predictive Coding Networks. In Proceedings of the 39th International Conference on Machine Learning (pp. 6793–6810). http://hdl.handle.net/20.500.12708/187543
- Predictive Coding beyond Gaussian Distributions / Pinchetti, L., Salvatori, T., Yordanov, Y., Millidge, B., Song, Y., & Lukasiewicz, T. (2022). Predictive Coding beyond Gaussian Distributions. In S. Koyejo, S. Mohamed, & A. Agarwal (Eds.), Advances in Neural Information Processing Systems 35 (NeurIPS 2022) (pp. 1280–1293). http://hdl.handle.net/20.500.12708/192691
- Learning on Arbitrary Graph Topologies via Predictive Coding / Salvatori, T., Pinchetti, L., Millidge, B., Song, Y., Bao, T., Bogacz, R., & Lukasiewicz, T. (2022). Learning on Arbitrary Graph Topologies via Predictive Coding. In Advances in Neural Information Processing Systems 35 (NeurIPS 2022) (pp. 38232–38244). Neural information processing systems foundation. http://hdl.handle.net/20.500.12708/192475
- Beyond Distributional Hypothesis: Let Language Models Learn Meaning-Text Correspondence / Jang, M., Mtumbuka, F., & Lukasiewicz, T. (2022). Beyond Distributional Hypothesis: Let Language Models Learn Meaning-Text Correspondence. In Findings of the Association for Computational Linguistics: NAACL 2022 (pp. 2030–2042). Association for Computational Linguistics. https://doi.org/10.18653/v1/2022.findings-naacl.156
Theses
-
Multimodal modeling of chest X-rays
/
Blasko, D. (2024). Multimodal modeling of chest X-rays [Diploma Thesis, Technische Universität Wien]. reposiTUm. https://doi.org/10.34726/hss.2025.118782
Download: PDF (1.76 MB) -
Automatically testing the out-of-distribution reasoning capabilities of LLMs/AGI systems with generative formal games
/
Vonderlind, P. (2024). Automatically testing the out-of-distribution reasoning capabilities of LLMs/AGI systems with generative formal games [Diploma Thesis, Technische Universität Wien]. reposiTUm. https://doi.org/10.34726/hss.2024.118784
Download: PDF (1.32 MB) -
Deep learning für das Semantic Web
/
Hohenecker, P. (2016). Deep learning für das Semantic Web [Diploma Thesis, Technische Universität Wien]. reposiTUm. https://doi.org/10.34726/hss.2016.37489
Download: PDF (1.11 MB)
Awards
-
Bayar Ilhan Menzat:
Outstanding Paper Award
2024 / Empirical Methods in Natural Language Processing / USA / Website
And more…
Soon, this page will include additional information such as reference projects, conferences, events, and other research activities.
Until then, please visit Artificial Intelligence Techniques’ research profile in TISS .