Best Paper Award at UCC 2023
Shashikant Ilager and his colleagues won best paper for their research on cloud data centers.
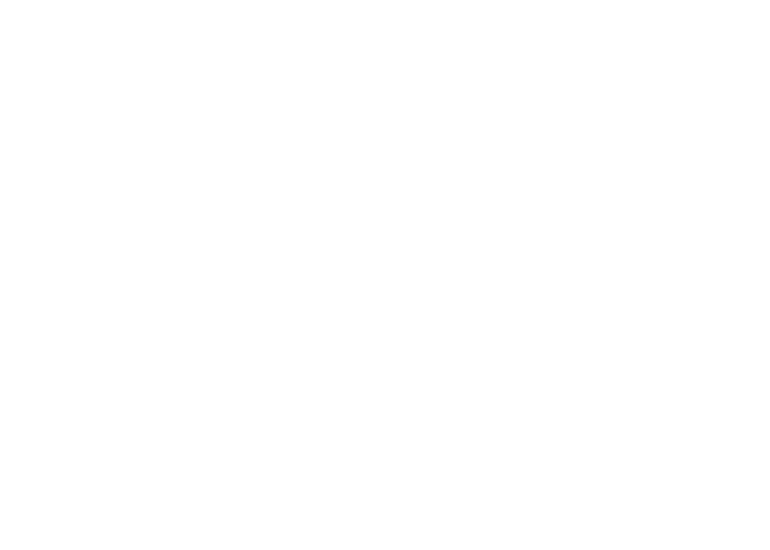
Shashikant Ilager, Mayank Raj Jha, Ivona Brandic, and their colleagues from Monash University and the University of Melbourne have won the Best Paper Award at the premier annual conference on Utility and Cloud Computing, the 16th IEEE/ACM UCC in Taormina, Italy from December 4–7, 2023.
Their paper A Data-driven Analysis of a Cloud Data Center: Statistical Characterization of Workload, Energy and Temperature was honored for its outstanding research contributions.
The research is founded on the fruitful cooperation between Mayank Raj Jha, who was a master’s student at TU Wien Informatics, and Shashikant Ilager, who co-supervised him. Mayank performed the first statistical analysis of the data center traces during his master’s thesis in the course of the joint master’s program MathMods Mathematical Modelling in Engineering: Theory, Numerics, Applications. The team has continued and intensified these efforts led by Shashikant Ilager.
Abstract
To efficiently manage large-scale cloud data centers, it is critical to understand data centers’ workload, energy, and thermal characteristics and their impact on the system through data-driven analysis. However, most publicly available traces solely focus on application workloads, ignoring energy and thermal aspects, forcing existing studies to rely on inaccurate and unrealistic analytical or simulation models. In this paper, we present a comprehensive data-driven analysis of a production cloud data center. We monitor and collect the physical machine-level metrics such as resource utilization, energy, and temperature for up to nine months, with a system size of over 26000 CPU cores hosting, on average 1300 virtual machines. We perform a systematic statistical analysis to characterize the monitored data and study their distributions, variations, trends, and inter-dependencies. We also develop data-driven models to predict resource usage and energy consumption of a physical machine and demonstrate the usefulness of our dataset through this use case. Our study reveals interesting insights into the energy and thermal phenomena of a data center. The outcome of this analysis helps to increase infrastructure efficiency and long-term strategic planning and improve key business KPIs. The ope-sourced dataset and artefacts enable to investigate new optimization approaches and use cases by researchers.
Curious about our other news? Subscribe to our news feed, calendar, or newsletter, or follow us on social media.