Assistant Professor with Tenure Track of ML for Cyber-Physical Systems
We invite applications for a full-time assistant professorship with tenure track in Machine Learning for Cyber-Physical Systems until August 7, 2025.
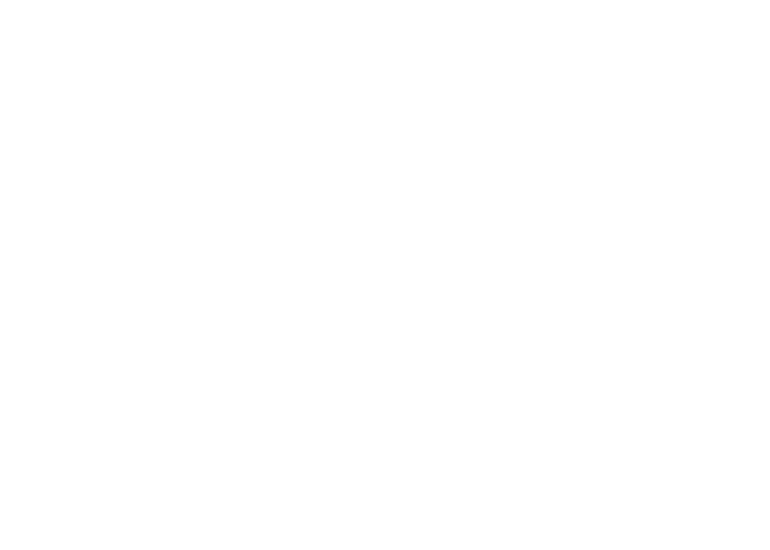
On This Page
About
TU Wien is Austria’s largest institution of research and higher education in the fields of technology and natural sciences. With over 26,000 students and more than 4000 scientists, research, teaching, and learning dedicated to the advancement of science and technology have been conducted here for more than 200 years, guided by the motto “Technology for People”. As a driver of innovation, TU Wien fosters close collaboration with business and industry and contributes to the prosperity of society.
The Faculty of Informatics, one of the eight faculties at TU Wien, plays an active role in national and international research and has an excellent reputation. The main areas of research include Computer Engineering, Logic and Computation, Visual Computing & Human-Centered Technology, as well as Information Systems Engineering.
The TU Wien Faculty of Informatics seeks to fill the open tenure-track position of an Assistant Professor of Machine Learning for Cyber-Physical Systems. The position is affiliated with the Institute of Computer Engineering, Research Unit Cyber-Physical Systems. The estimated starting date is March 2026. The work contract is initially limited to six years. The candidate and TU Wien can agree upon a tenure evaluation, which when positive, opens the possibility to change the position to Associate Professor with an unlimited contract.
Focus
Cyber-physical systems (CPS) and the Internet of Things (IoT) are the foundational software/hardware systems for strategically essential fields in modern society. These sectors include self-driving cars, autonomous robots, Industry 4.0, smart agriculture, smart grids, intelligent buildings, and advanced healthcare. Due to the growing use and the severe consequences that can arise from the potential malfunction of CPS/IoT systems, the modelling, analysis, and optimal control of CPS/IoT systems have become critical societal concerns. Given the complexity inherent in these fields, traditional first-principle approaches need to be enhanced with modern, data-driven methodologies. Stochastic machine-learning techniques, in particular, will be essential for CPS/IoT systems, as they not only help extract insights from the vast amounts of data generated by CPS/IoT systems but also take advantage of the stochastic nature of these data. Supervised, unsupervised, and reinforcement learning methods have already shown significant potential for model inference, predictive accuracy, and optimal control within these fields. It is crucial to scale these methods for large-scale CPS/IoT applications, such as smart cities, and to improve the reasoning about their high-level data features. This improvement promises to establish a stochastic foundation for a cognitive understanding within these systems. Additionally, lifelong learning has been recognized as an essential component of future cognitive CPS/IoT systems. This requires robust mathematical foundations and lightweight designs for edge devices.
The research areas of the successful candidate should cover some of the following research topics for CPS/IoT, but are not limited to them:
- Deep Neural Networks, Residual Networks, Neural Ordinary Differential Equations (ODEs)
- Unsupervised Learning, Autoencoders, Generative Adversarial Networks (GANs)
- Supervised Learning, Vanishing Gradients, Degradation Problems
- Sim2Real Reinforcement Learning methods
- Testing and shielding of reinforcement learning agents
- Multi-agent Reinforcement Learning
- Monitoring Deep Neural Networks and Out-of-Distribution detection
- Attention Mechanisms, Transformers, Higher-Order Reasoning in Deep Neural Networks, Structured State Space Models, Mixture of Experts
- Large Language Models with applications in system design and requirement engineering of CPS
- Explainable AI
- Neuro-Symbolic AI
Applicants must have a strong expertise and research record in Machine Learning for CPS/IoT. The candidate is expected to have published in the last four years their work as a regular paper in top-tier conferences and journals. The candidate should have relevant postdoctoral experience and at least one year of research or study experience outside of Austria. A compelling research vision is essential.
Besides research, the duties of an Assistant Professor at TU Wien include graduate and undergraduate teaching (in English and German), supervision of student work, and contributing to usual management and faculty service tasks. Potential collaborations within the institute, the faculty, and the university are highly encouraged. Independence in conducting research is crucial, and experience in acquiring competitive grants will be considered a significant asset. Additionally, international embedding in the research field is essential.
Application
For additional details and to enter the application process, please see the TU Wien Job Platform:
Curious about our other news? Subscribe to our news feed, calendar, or newsletter, or follow us on social media.