#5QW: Emanuel Sallinger
“I like seeing how teaching and research come together and applying that to the real world.”
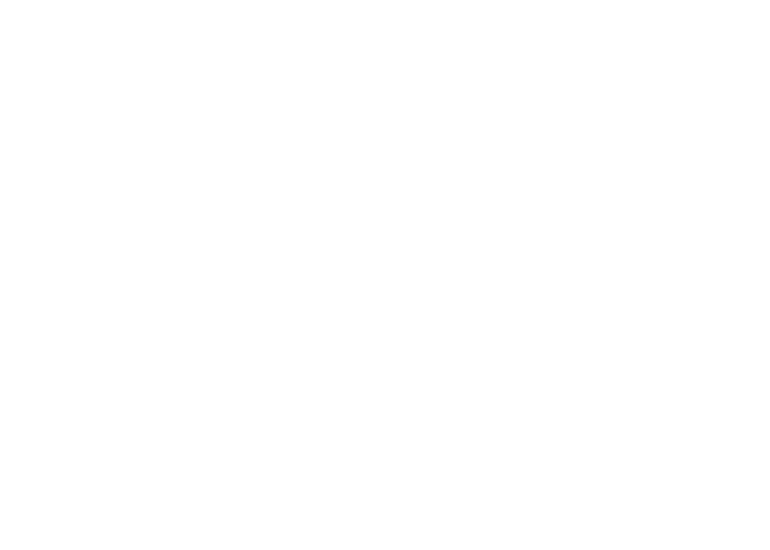
Picture: TU Wien Informatics + AI
How would you describe your work in 90 seconds?
My research is in Data Management and Artificial Intelligence, and there are two key points that I focus on. One is scalability and the second is broadness. Scalability in computer science often means making things faster, but that’s only one important aspect. We do not just want answers from AI black boxes, but we also want to know how an AI system comes to an answer; we want explanations. The question is, can AI systems scale with larger and larger data that you put into them so that humans can still make use of the explanations? Then, we also need our AI systems to be sustainable; in other words, can AI systems actually scale down to use fewer resources and still offer good results? Another aspect of my work revolves around complexity, in what is called Knowledge Graphs, which is one of my primary research areas; the question here is if AI systems still scale with large and complex graph structures. However, scalability alone is not enough, we also need broadness. In AI, there are usually three parts: One of them is Data Management, which includes data and knowledge engineering to put the right data into the AI system. The second and third are often called sub-symbolic AI, or Machine Learning including LLMs, and symbolic AI, which includes logical reasoning. Getting all three of these parts to work together is not easy, but it’s crucial. Recently, the Austria-wide Cluster of Excellence “Bilateral AI” was launched on getting symbolic and sub-symbolic to work together. Getting scalable, broad AI systems is at the center of my work.
How did you get in touch with informatics?
Like many others, my interest in computer science was triggered by my family and school. There are two interesting facts regarding that which come to mind: The first is that I went to the same Gymnasium (ed. note: a form of secondary school) as one of Europe’s computing pioneers, Heinz Zemanek, namely the BRG Marchettigasse. Zemanek built the first fully transistor-based computer in continental Europe, the so-called Meilüfterl, or ‘spring breeze’. The second one is that for high school, I went to HTL Spengergasse, which is a type of high school where one gets deeply exposed to technical topics, such as computer science. At the time, things like Machine Learning and AI were not really popular. You could hear things like, will these artificial neural networks and logic ever be useful for something? I had teachers who actively worked with us on AI, logic, and neural networks, and that’s a crucial time to be inspired by that when you’re 15,16 years old.
Where do you see the connection between your research and everyday life?
One good example is our recent project on AI and sustainable waste collection, processing, and recycling. The Vienna Science and Technology Fund, WWTF, has a project line that looks at how science translates into real-world positive impact. We all have to think about how to separate organic waste from other types of waste, pretty much daily, and we know that errors can happen when you’re separating waste. The worst-case scenario (and hopefully, that doesn’t happen to a lot of us frequently) is if you put a leaky battery into your organic waste, not only will your own organic waste be contaminated, but the entire load of the waste truckm and so making recycling impossible. This may seem simple at first glance, but it’s quite challenging, especially if you look at it at the level of a district, a city, or a whole country. One of the most interesting challenges is how to use AI to balance CO2 emissions and fine dust pollution that garbage trucks produce versus the recycling quota, but AI is used on multiple levels. First, we have visual processing, so that an AI system can recognize an apple here and a leaky battery there. If you take the metaphor of brain lateralization and fast and slow thinking, this is the fast-thinking part, the neural networks. The second way that AI is used is for planning. Say we have 20,000 points where we need to collect waste, so then we need to find routes for the garbage trucks to do that. If the collected waste at one particular pick-up point is always contaminated, maybe we don’t include it in the route because there are always leaky batteries in that load. That’s the slow thinking, the AI that is more about planning and logic-based reasoning based on graph structures. This is a good example of bilateral AI (ed. note: combining symbolic and sub-symbolic AI), because we need both the fast thinking, aha, this is a battery, and we need the slow thinking, okay, how do we now plan this route to create as little as possible CO2 emissions?
What makes you happy in your work?
Working on fascinating problems; that’s an answer you probably hear quite often (laughs). However, there are two other things I enjoy a lot, and one of them is teaching. It’s one thing to research interesting topics, but teaching requires a lot of organization to make a topic as clear and well thought-through as possible, and you also want it to be interesting to students. So, you need to find ways to make it exciting as well. I think it takes a lot of effort to understand a topic at a level so that you can teach it well and find ways so that it becomes exciting, because depending on how you present it, it can also become boring. Also, it’s reasonably easy to teach according to a textbook, but when you bring it down to essential concepts, so-called threshold concepts, it’s a lot of work, because those concepts are the hardest ones to grasp. The other thing that I enjoy about my work is translating my research into practice for companies and organizations. For example, we have a longstanding collaboration with the Central Bank of Italy, where we develop anti-money laundering techniques, and ways to protect organizations and economic systems from hostile attacks. I think you can also see that it is connected to everyday life in many ways. So, I also like seeing how teaching and research come together and applying that to the real world.
Why do you think there are still so few women in computer science?
This is a complex question, and one thing that is clear is that we need to push this issue more. The key is to start early to make computer science a fascinating and exciting area for both girls and boys at a point where there aren’t a lot of preconceived notions and stereotypes yet. One program that I would really like to mention here is TU Wien Informatics eduLAB, which brings computer science education to schools with workshops ranging from elementary school up to high school. The workshops are held for girls and boys together, and students can playfully explore topics like algorithms, coding, or Artificial Intelligence. It’s really something that one needs to see to understand how big the impact is. This also brings me back to the question of what one enjoys: It’s important to organize topics in a way that they’re not only correct; that’s one thing, but they also need to be presented well and fascinating to students. That is true for students at an elementary school, middle school, or high school level. So, simply put, making computer science a lot of fun early on is a good starting point, in my view.
Emanuel Sallinger is Professor of Databases and Artificial Intelligence at TU Wien Informatics. Emanuel Sallinger will hold a joint Inaugural Lecture on October 15, 2024; 17:00 CEST at EI 9, Gußhausstraße together with Magdalena Ortiz and Dominique Schröder.
Curious about bilateral AI? Learn more about the work of the Cluster of Excellence BILAI.
Discover the whole #5QW series.
Curious about our other news? Subscribe to our news feed, calendar, or newsletter, or follow us on social media.