#5QW: Matthias Lanzinger
“At university, there’s a lot of freedom and room for creativity to express what’s on your mind.”
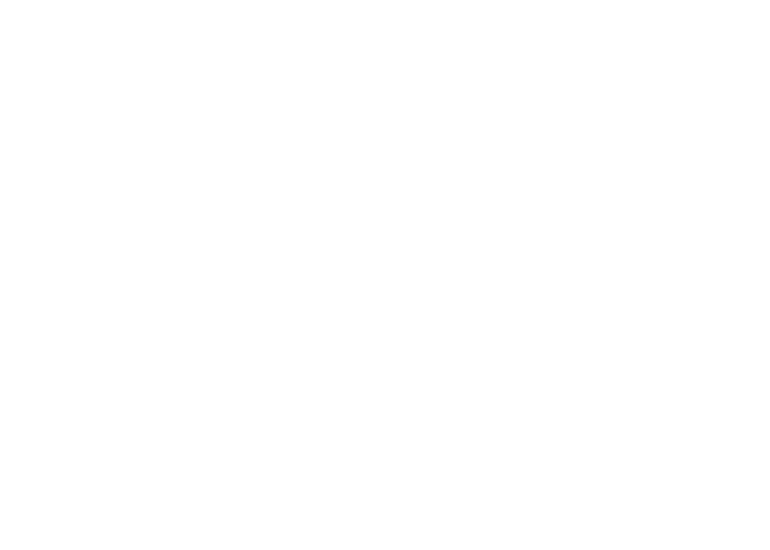
Picture: TU Wien Informatics
How would you describe your work in 90 seconds?
I study the limits of what computers can do or the limits of what systems on computers can do. In the past, I’ve mostly worked on the limit of languages with which you can access data. If you have a language with which you access your data, there are some boundary problems, something you can’t describe in this language, and problems that are too difficult or too complex. A lot of work goes into understanding these boundaries, and we can determine these boundaries very precisely. In computer science, we have tools to do this through mathematical proof to see exactly what is possible, and what is not. More recently, we started to do this for Machine Learning (ML) systems, where you want to know what kind of concepts they can learn. Now, we can also see where the boundaries are for a wide range of systems, particularly for graph neural networks, which are used to learn about molecules, protein networks, or traffic networks. This approach is very useful for understanding the structure of networks, and to understand which properties of networks can be learned by such a system. We can, in a mathematically precise way, say how much expressivity a specific type of system has so we can determine whether a concept falls within this boundary or outside of it. If it falls outside, then the system is fundamentally too weak to learn about the properties of the network, and there’s no way that it could ever learn this. If it falls within the boundary, we have at least a theoretical guarantee that it’s possible for the system to learn, so there is some way in which it can learn about the properties of the network. I look into these boundaries of what is possible and what isn’t, with different types of systems.
How did you get in touch with informatics?
Very early on. When I was eight or nine, I think, the first home computers and the internet became very popular and widespread. We had a computer at home, and I think from then on, I started getting interested in computer science and never stopped. The computer we had was standing on a desk and used by the whole family, which was very common at the time. I did a lot of things; I started editing video games, I started looking into how different parts work, and I started changing things, changing how it works. From there, I got further into programming, and over time, it got more and more elaborate, diving in to see how things work. In my first year of studying computer science, there was an introductory course to theoretical computer science, and with this course, it just instantly clicked for me. Since then, I have been fully immersed in theoretical computer science.
Where do you see the connection between your research and everyday life?
My research is more on the theoretical and abstract side (laughs). But I wouldn’t say that it doesn’t touch on everyday life, it’s just the path is longer and more indirect. A lot of what I do is related to the foundations on which you can build work that goes from theory to practice. For example, there are database systems that are running in the background of every website, and these systems have a lot of responsibility. They have a lot of things they need to be able to do, and you need to design these systems in a way in which you can understand how they work; for that, you need to go back to the theoretical foundations from the 70s and 80s. Now, we’re building new foundations, but these new foundations will form the basis for more advanced performance systems in the next ten to twenty years. In that way, what I do is more indirect, and with ML systems, the life cycle is faster because everything happens very fast. It’s also important to understand what the limits of these systems are and why they are there. By understanding these limits, we can also push these limits. We’ve introduced methods of how to push these boundaries in a targeted way, so that they capture specific things that you know are not in the system yet. So, what I do has to do is foundational research, but we’ve even shown in experiments that this approach works, and I hope that in the coming years, this will propagate into more practical applications. My advice to anyone who’s looking to get into ML would be to not be as narrow as to start in ML only. Try to start with something that you find interesting, something with which you can then build a bridge to ML. Likely, this will be much more productive and make for much more interesting research, and you also have to be careful not to get too caught up in research trends. In Computer Science, you can create things; you decide what they look like and how they operate.
What makes you happy in your work?
Many, many things - I think the first that comes to mind is freedom. Not just logistically in what I do day to day, but on the very theoretical side of things. Normally, you wouldn’t take something someone has done and iterate on it so much. At university, you have the freedom to come up with completely new things, and in a way, sometimes you just come up with new parts of mathematics for what you want to do. There’s a lot of freedom and room for creativity to express what’s on your mind. You can try things out, and if it makes sense, you can convince people that it’s a good thing, even if you do something completely new. So, I really enjoy that you’re not so restricted in terms of the directions that you can go in. Also, to me, the problems I get to think about are just very interesting, and it’s nice to have the time to think about problems properly. Between my master’s and my PhD, I worked in the private sector for a few years and that was a very different experience. It was typical in that you have some tasks and problems to solve, but you don’t have weeks to sit around and think about different ways to do this. You don’t actually have the time to sit around and think. At university, I can take, say, two weeks to think about this one specific thing. And this time to think about things, I appreciate that very much, it makes thinking much more enjoyable to me. If you’re in a rush, you can’t do proper thinking.
Why do you think there are still so few women in computer science?
This is very difficult to say nicely. I think that at least in Austria, it’s a big societal problem. I’ve seen the efforts that TU and the faculty are making, and they’re very proactive, but I’m sure that the university and faculty can and should do more still. I see how a lot of the initiatives have very muted responses, and you see the gender ratio improving very slowly for undergraduates, and it only balances out in master’s and the PhD programs. To me, that is indicative of larger societal issues, like the feedback or direction people are pushed in in school. Growing up in Austria and speaking of my own experience, I do think that these problems are systemic. I don’t know how much has changed, but I do think that there’s still an element of societal pressure. That, to me, is a bigger issue, a more fundamental issue. Individual efforts and the efforts of the faculty are great, but they are only addressing the symptoms because it’s hard for universities to address the underlying causes if they are completely outside of their scope. At the faculty, many people are working very hard on this problem, and they’re very smart people, so it’s obviously very difficult, and people are trying very hard to improve the status quo.
Matthias Lanzinger is Assistant Professor at the Research Unit Databases and Artificial Intelligence at TU Wien Informatics. He is Co-Principal Investigator on the project Decompose and Conquer: Fast Query Processing via Decomposition. The project is funded by the WWTF and will run until 2026.
Discover the whole #5QW series.
Curious about our other news? Subscribe to our news feed, calendar, or newsletter, or follow us on social media.