Inaugural Lectures in October
TU Wien Informatics is pleased to welcome our new professors. Magdalena Ortiz, Emanuel Sallinger, Dominique Schröder will present their exciting work.
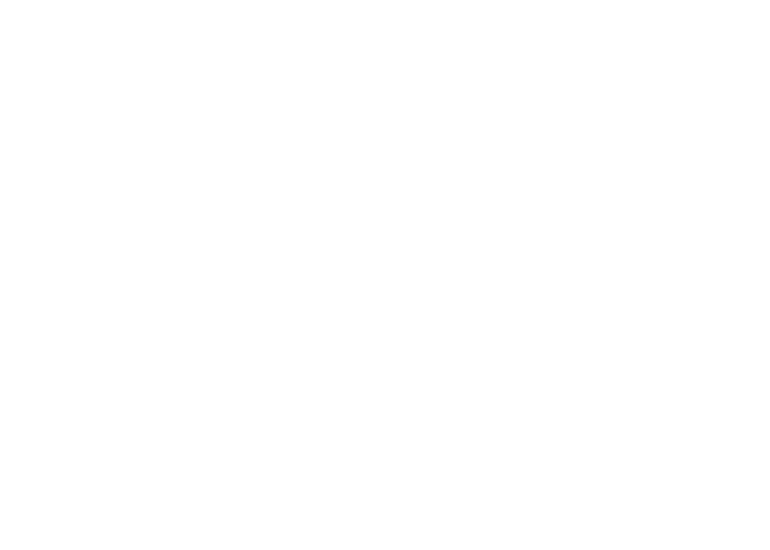
- –
-
TU Wien, Campus Gußhaus
EI 9 Hlawka-Hörsaal -
1040 Vienna, Gußhausstraße 27-29
Stiege 1, Erdgeschoß, Raum CAEG17
We are pleased to welcome our new professors who joined the Faculty of Informatics. Magdalena Ortiz, Emanuel Sallinger, and Dominique Schröder will present their exciting work. Don’t miss this opportunity to meet them in person and learn more about their exciting fields of work!
Programm:
The event will be moderated by the Dean of TU Wien Informatics Gerti Kappel.
Inaugural lectures by:
We would like to invite you to a reception with food and drinks at the end of the event. If you’re curious to learn more about our new Professors before their Inaugural Lecture, check out our interview series #5QW Magdalena Ortiz, #5QW Emanuel Sallinger and #5QW Dominique Schröder.
Magdalena Ortiz
Title
Pushing the boundaries of rational AI
Abstract
One of the most ambitious and exciting ideas that has driven AI since its early days is the explicit representation of human knowledge and the drawing of inferences from it by means of automated reasoning. We are witnessing a new AI era where large-scale learning from vast quantities of data has enabled the simulation of increasingly sophisticated human-like behaviors. But despite all the recent progress, knowledge-based rational inference remains very hard to reproduce in machines. Due to the subtle intricacies of human reasoning and the high computational cost of inference from vast amounts of knowledge, there are still many challenges to achieving a transparent and accurate AI that can be trusted even in unforeseen circumstances, and that can be explained in human-understandable terms. The field of Knowledge Representation and Reasoning has developed a rich toolkit of techniques that enable rational inference, leveraging different types of knowledge, accommodating various domain assumptions, and supporting increasingly complex information needs. As we better understand different requirements and gain a better grasp of the fine balance between the expressiveness of languages and the cost of computation, we can now provide tailored solutions that support the desired decision-making at a minimal computational cost, making it feasible to build increasingly intelligent systems: An AI that does not require extensive training to improve its ability to make accurate predictions, as it can logically reach correct conclusions.
Emanuel Sallinger
Title
Scalable AI: From Scalable Data Management to Neurosymbolic AI
Abstract
Scalability in computer science is often associated with speed. Speed is one important aspect of Artificial Intelligence, but not the only one. We do not only want answers from our AI systems, but we also want explanations of these answers. With growing input sizes, can explanations scale so that they remain useful to us as humans? Second, we need our AI systems to be sustainable – so scale down the resource usage and still give good results. Third, we need AI systems to scale with growing complexity – in my research related to Knowledge Graphs, this is often associated with complexity measures of large graphs and expressive knowledge. Solving these challenges individually is not enough. We need AI systems that jointly scale on all their core components. On the one hand, this is scalable data management, allowing effective access to data. On the other hand, this is joint scalability in the two main AI families: sub-symbolic AI – that is machine learning including LLMs – and symbolic AI – including logical reasoning. This combination is typically called bilateral or neurosymbolic AI. Getting all three parts closely working together is not easy, but it is crucial. In my lecture, I will give an overview of these aspects and illustrate them in one of my major areas of research, Knowledge Graphs, where we have a need for all the above: Extremely large real-world graphs with very complex knowledge, the need for clear explanations, and sustainability as a key concern and application at the same time. To achieve this, we need scalable graph data management and both sub-symbolic AI methods such as Knowledge Graph Embeddings, Graph Neural Networks, and LLMs – and symbolic AI methods such as Datalog- and Vadalog-based reasoning. I will give real-world examples of my work in these areas, like the work with our partners at the Central Bank of Italy, and an outlook and vision of what comes next at the intersection of data management, data science, and Artificial Intelligence.
Dominique Schröder
Title
Empowering Innovation: Unlocking the Potential of Privacy-Enhancing Technologies
Abstract
Data collection is growing rapidly due to the increasing number of connected devices, from smartphones to smart home systems, and advances in artificial intelligence that make data processing more efficient. Businesses and governments are collecting data to enhance decision-making, improve services, and create personalized experiences. For example, smart healthcare can monitor patients remotely, connected devices can optimize energy use, and smart cities can reduce traffic congestion. However, this data collection raises privacy concerns, such as the risk of sensitive health data being exposed, devices recording conversations without consent, and personal data being shared with third parties. Privacy is often seen as a problem or unnecessary because of common prejudices. One is the belief that “I have nothing to hide,” which leads people to dismiss privacy concerns as irrelevant to them personally. Another misconception is that privacy hinders technological progress, with some seeing it as an obstacle to innovation in areas such as AI, smart devices, or personalized services. In this talk, I will address both misconceptions. First, I will show how even small pieces of information can uniquely track and identify individuals, proving that privacy concerns affect everyone, not just those with “something to hide”. Second, I will show how privacy-enhancing technologies enable modern advances without violating individual privacy. From encrypted communications to differential privacy in data analysis, these tools allow us to innovate while protecting personal information at the same time, proving that privacy and technological progress can coexist.
Curious about our other news? Subscribe to our news feed, calendar, or newsletter, or follow us on social media.